by Scott Muniz | Aug 18, 2020 | Azure, Microsoft, Technology, Uncategorized
This article is contributed. See the original author and article here.

In a historic day, Microsoft today announced it has transitioned Azure HDInsight to the Microsoft engineered distribution of Apache Hadoop and Spark, specifically built to drastically improve the performance, improved release cadence of powerful Open Source data analytics frameworks and optimized to natively run at cloud scale in Azure. This transition will further help customers by establishing a common Open Source Analytics distribution across various Azure data services such as Azure Synapse & SQL Server Big Data Clusters.
Starting this week, customers creating Azure HDInsight clusters such as Apache Spark, Hadoop, Kafka & HBase in Azure HDInsight 4.0 will be created using Microsoft distribution of Hadoop and Spark.
As part of today’s release, we are adding following new capabilities to HDInsight 4.0
SparkCruise: Queries in production workloads and interactive data analytics are often overlapping, i.e., multiple queries share parts of the computation. These redundancies increase the processing time and total cost for the users. To reuse computations, many big data processing systems support materialized views. However, it is challenging to manually select common computations in the workload given the size and evolving nature of the query workloads. In this release, we are introducing a new Spark capability called “SparkCruise” that will significantly improve the performance of Spark SQL.
SparkCruise is an automatic computation reuse system that selects the most useful common subexpressions to materialize based on the past query workload. SparkCruise materializes these subexpressions as part of query processing, so you can continue with their query processing just as before and computation reuse is automatically applied in the background — all without any modifications to the Spark code. SparkCruise has shown to improve the overall runtime of a benchmark derived from TPC-DS benchmark queries by 30%.
SparkCruise: Automatic Computation Reuse in Apache Spark
Hive View: Hive is a data warehouse infrastructure built on top of Hadoop. It provides tools to enable
data ETL, a mechanism to put structures on the data, and the capability to query and analyze large data sets that are stored in Hadoop. Hive View is designed to help you to author, optimize, and execute Hive queries. We are bringing Hive View natively to HDInsight 4.0 as part of this release.
Tez View: Tez is a framework for building high-performance batch and interactive data processing applications. When you run a job such as a Hive query Tez, you can use Tez View to track and debug the execution of that job. Tez View is now available in HDInsight 4.0
Frequently asked questions
What is Microsoft distribution of Hadoop & Spark (MDH)?
Microsoft engineered distribution of Apache Hadoop and Spark. Please read the motivation behind this step here
• Apache analytics projects built, delivered, and supported completely by Microsoft
• Apache projects enhanced with Microsoft’s years of experience with Cloud-Scale Big Data analytics
• Innovations by Microsoft offered back to the community
What can I do with HDInsight with MDH?
Easily run popular open-source frameworks—including Apache Hadoop, Spark, and Kafka—using Azure HDInsight, cost-effective, enterprise-grade service for open-source analytics. Effortlessly process massive amounts of data and get all the benefits of the broad open-source ecosystem with the global scale of Azure.
What versions of Apache frameworks available as part of MDH?
Component
|
HDInsight 4.0
|
Apache Hadoop and YARN
|
3.1.1
|
Apache Tez
|
0.9.1
|
Apache Pig
|
0.16.0
|
Apache Hive
|
3.1.0
|
Apache Ranger
|
1.1.0
|
Apache HBase
|
2.1.6
|
Apache Sqoop
|
1.4.7
|
Apache Oozie
|
4.3.1
|
Apache Zookeeper
|
3.4.6
|
Apache Phoenix
|
5
|
Apache Spark
|
2.4.4
|
Apache Livy
|
0.5
|
Apache Kafka
|
2.1.1
|
Apache Ambari
|
2.7.0
|
Apache Zeppelin
|
0.8.0
|
In which region Azure HDInsight with MDH is available?
HDInsight with MDH is available in all HDInsight supported regions
What version of HDInsight with MDH will map to?
HDInsight with MDH maps to HDInsight 4.0. We expect 100% compatibility with HDInsight 4.0
Do you support Azure Data Lake Store Gen 2? How about Azure Data Lake Store Gen 1?
Yes, we support storage services such as ADLS Gen 2, ADLS Gen1 and BLOB store
What happens to the existing running cluster created with the HDP distribution?
Existing clusters created with HDP distribution runs without any change.
How can I verify if my cluster is leveraging MDH?
You can verify the stack version (HDInsight 4.1) in Ambari (Ambari–>User–>Versions)
How do I get support?
Support mechanisms are not changing, customers continue to engage support channels such as Microsoft support
Is there a cost difference?
There is no cost or billing change with HDInsight with Microsoft supported distribution of Hadoop & Spark.
Is Microsoft trying to benefit from the open-source community without contributing back?
No. Azure customers are demanding the ability to build and operate analytics applications based on the most innovative open-source analytics projects on the state-of-the-art enterprise features in the Azure platform. Microsoft is committed to meeting the requirements of such customers in the best and fastest way possible. Microsoft is investing deeply in the most popular open-source projects and driving innovations into the same. Microsoft will work with the open-source community to contribute the relevant changes back to the Apache project itself.
Will customers who use the Microsoft distribution get locked into Azure or other Microsoft offerings?
The Microsoft distribution of Apache projects is optimized for the cloud and will be tested extensively to ensure that they work best on Azure. Where needed, the changes will be based on open or industry-standard APIs.
How will Microsoft decide which open-source projects to include in its distribution?
To start with, the Microsoft distribution contains the open-source projects supported in the latest version of Azure HDInsight. Additional projects will be included in the distribution based on feedback from customers, partners, and the open-source community.
Get started
by Scott Muniz | Aug 18, 2020 | Azure, Microsoft, Technology, Uncategorized
This article is contributed. See the original author and article here.
Azure Sphere OS version 20.08 Update 1 is now available in the Retail Eval feed. This OS release contains a security update.
Because this change represents a critical update to the OS, the retail evaluation period will be shortened and the final 20.08 release will be published as soon as possible within the next 7 days to ensure systems stay secure. During this time, please continue verifying that your applications and devices operate properly with this retail evaluation release before it’s deployed broadly via the Retail feed. The Retail feed will continue to deliver OS version 20.07 until we publish the final 20.08 release.
For more information
For more information on Azure Sphere OS feeds and setting up an evaluation device group, see Azure Sphere OS feeds.
If you encounter problems
For self-help technical inquiries, please visit Microsoft Q&A or Stack Overflow. If you require technical support and have a support plan, please submit a support ticket in Microsoft Azure Support or work with your Microsoft Technical Account Manager. If you would like to purchase a support plan, please explore the Azure support plans.
by Scott Muniz | Aug 18, 2020 | Azure, Microsoft, Technology, Uncategorized
This article is contributed. See the original author and article here.
Over the last two months, I’ve engaged with a few media companies who wanted to learn how to integrate Azure Media Services into their workflow. Azure Media Services (AMS) offers a whole range of media capabilities for content ingest, storage, transcoding, video AI, encryption, dynamic packaging, and delivery – be it for live streaming or VOD through a single or multi-CDN.

This post contains 2 sample workflow implementations for content ingest and clear stream publishing
- A logic app that monitors newly uploaded content in an Azure Storage for transcoding and indexing.
- A logic app for publishing a media asset with a clear stream locator.
Reference and Set-up
Before I start, let me just share first that I referred to these 2 GitHub Repos extensively in implementing this logic app:
In doing so, I had to refactor some functions and split them into two. I also had to add some more functions to suit my needs. So I forked the v3 repository, which you can check out here: https://github.com/raffertyuy/media-services-v3-dotnet-core-functions-integration.
Create an Azure Function App and deploy the functions found in the advanced-vod-workflow folder.
Also, if you prefer to skip reading the logic app documentation below, feel free to clone the repo above and go straight to the code.
Upload and Process Workflow
Media companies will have an existing process for the creation of mezzanine files. These files are then typically uploaded to a CDN for content distribution. Given that this process already exists, the idea here is to add/modify the upload destination to an Azure Storage instead and expect it to be processed in Azure Media Services.
Media Service Assets
An Asset is a core concept specific to Azure Media Services (AMS). It is not a single blob in Azure Storage. An asset from a storage perspective will contain multiple files, such as its metadata, multiple bitrate files, etc. Internally, AMS handles this by mapping each asset to an Azure Blob container, which contains all of these files.
So the main challenge is how to upload a mezzanine file and get it recognized as a media asset by Azure Media Services, and this is where logic apps come in.
The Logic App
Azure Logic Apps is a cloud service that helps you schedule, automate, and orchestrate tasks, business processes, and workflows when you need to integrate apps, data, systems, and services across enterprises or organizations. In this particular case, we are using logic apps to:
- Monitor an Azure Storage account for new uploads
- Create an Asset in Azure Media Services
- Transcode the asset into multi-bitrate
- Send to Azure Video Indexer for Video AI insights
In implementing this logic app, I used a lot of connectors, including a connector to Azure Functions. I used Azure functions to execute code that is not available in Logic Apps.
Monitor an Azure Storage Account for New Uploads
This one is easy; I used the Azure Blob Storage trigger to monitor for new files uploaded to my /mezzanine
Azure storage container. But since media files usually are quite large, we need to ensure that the file is segmented in chunks. As recommended in this article, the way is to use the “properties only” trigger and then use an additional Get blob content action.
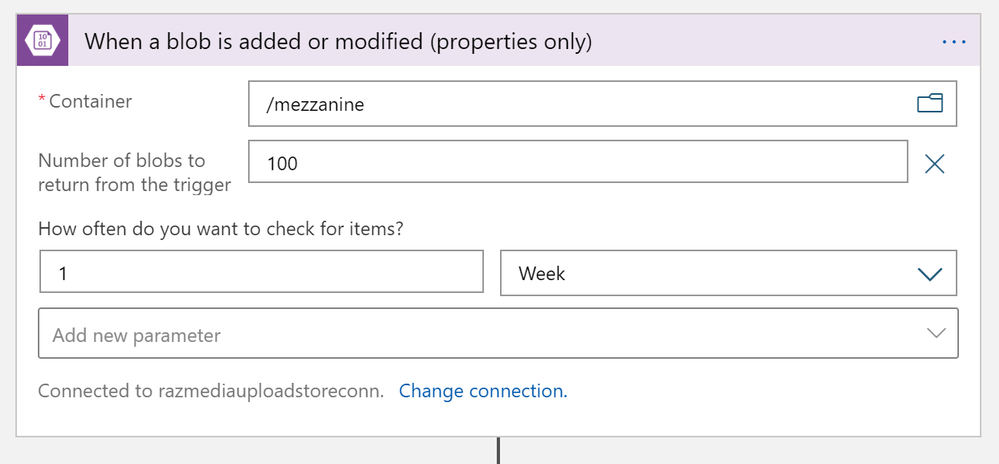
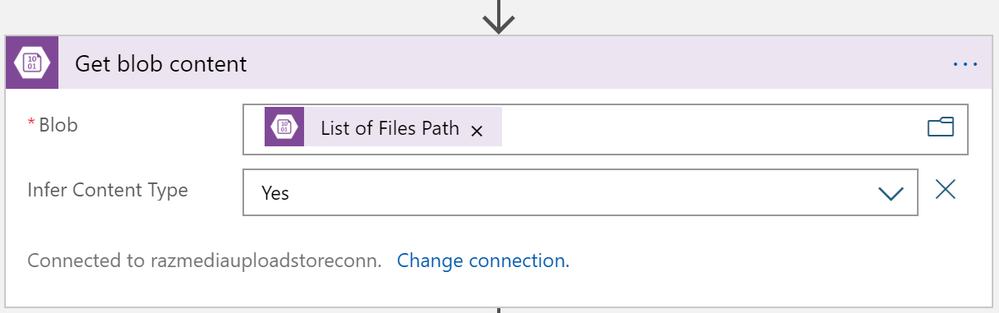
Create an Asset in Azure Media Services
Interestingly, AMSv3, at this point, does not have a quick API call to convert a file into a recognized media asset. The process is first to create an “empty asset” and then copy the blob content over. The process looks like this:
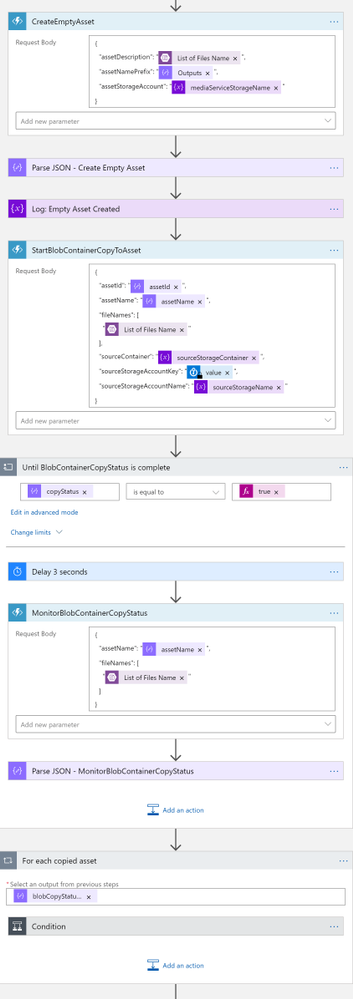
Note that CreateEmptyAsset, StartBlobContainerCopyToAsset, and MonitorBlobContainerCopyStatus are Azure Functions.
Transcode the Asset into Multi-bitrate
Azure Media Services has out-of-the-box presents for us to do our tests, but in a real-world scenario, a media company will likely have their custom-defined list of transcoding profiles. In this sample implementation, I used the H264MultipleBitrate1080
preset. I then created a Transform (if it doesn’t yet exist) and then submitted a job for transcoding. You may refer to this article to understand these concepts better.
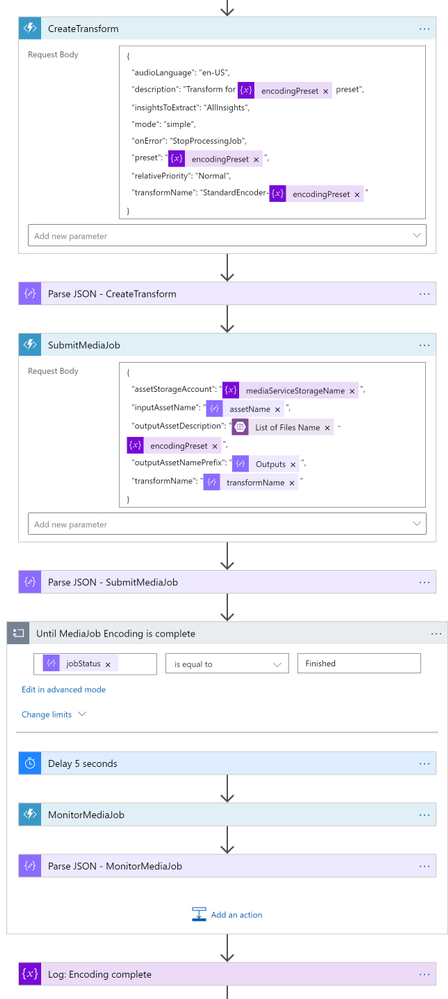
So I used the CreateTransform and SubmitMediaJob functions here. If you are planning to modify this logic app to suit your needs, you may need to spend some time understanding how to use these functions. In this logic app, this is where I spent most of my time. The sample code isn’t very well documented and not bug-free (As previously stated, this is a forked repo from AzureSamples), so it required a lot of trial-and-error and bug fixing.
If you are planning to create a new custom preset, you need to set "preset": "CustomPreset"
and then define a "customPresetJson": {...}
according to this.
After the asset is transcoded, I then move the asset to another blob container. Since there is no “move” action, I had to create in the destination container first, and then delete the original one.
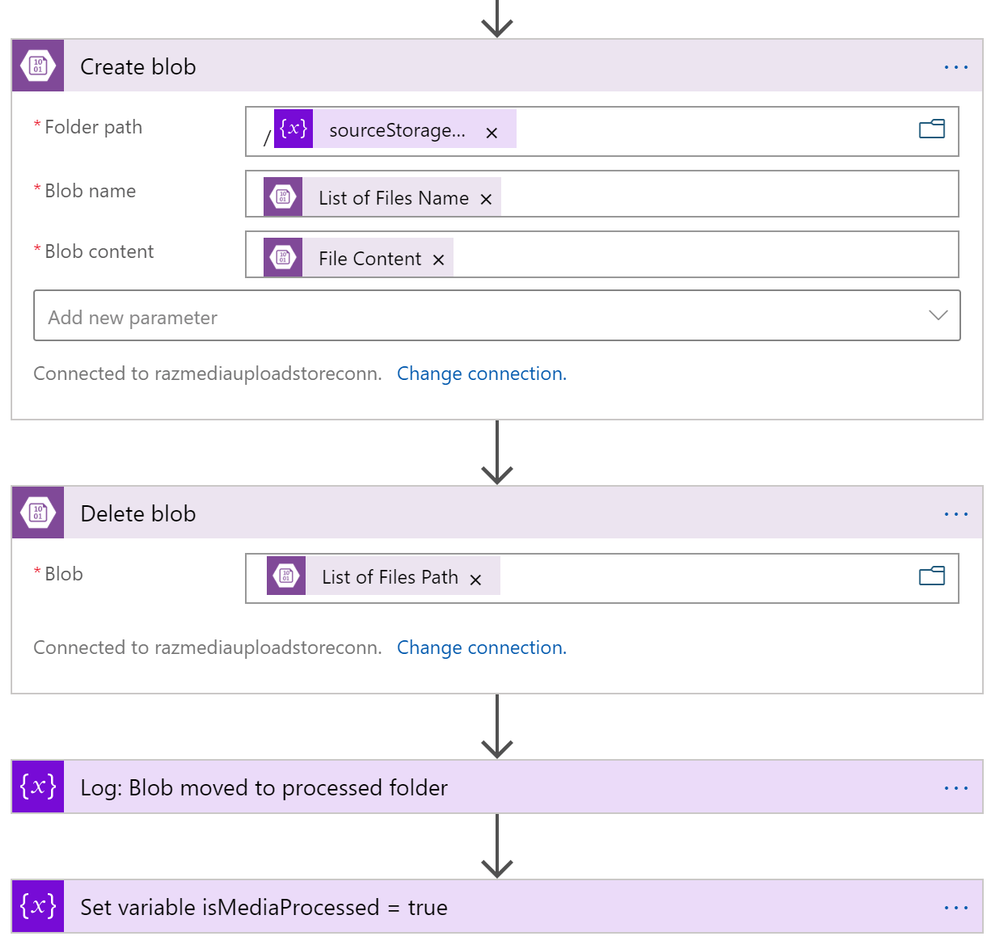
Send to Azure Video Indexer
Parallel to transcoding, I added another branch for sending the file to Video Indexer. Azure Video Indexer is the media service AI solution for extracting more insights such as the transcripts, keywords, sentiments, celebrities, landmarks, etc. that are found in the media asset.
In uploading, you may choose to upload from a blob URL straight. But since we already have a media asset, I went with the Asset ID parameter option.
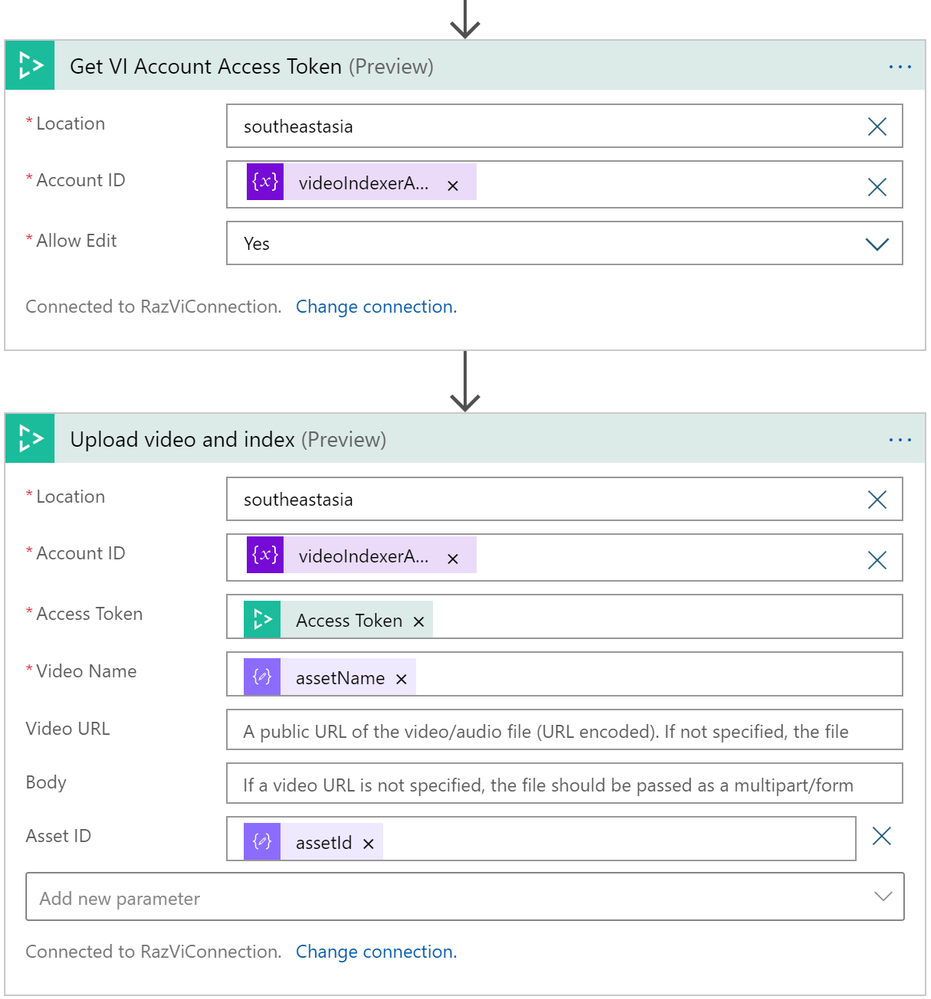
One interesting note here is that VI will transcode the asset again into a 720p Single Bitrate file.
Output
And here are the results after running this logic app
Azure Media Service Explorer
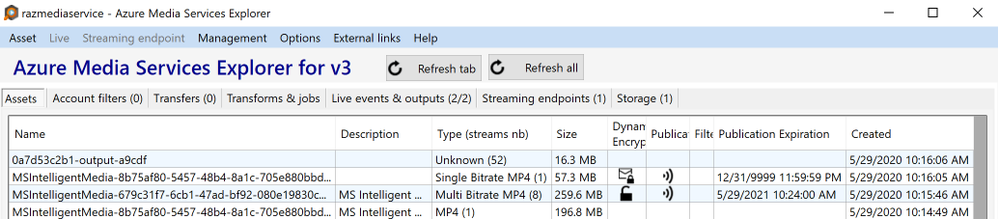
Media Asset in the Azure Storage
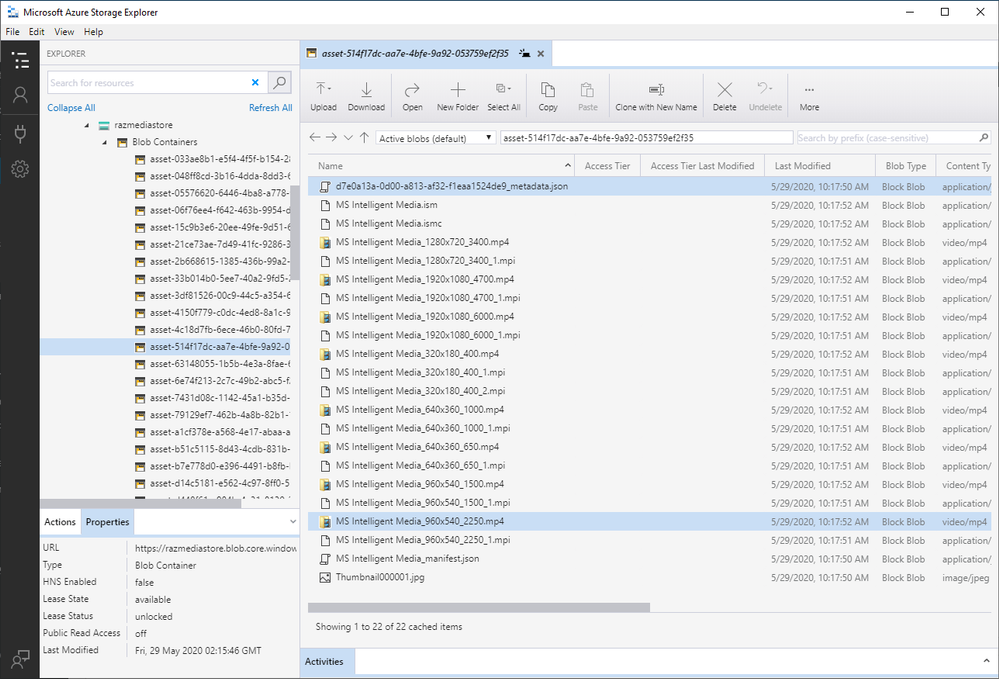
Insights from Azure Video Indexer
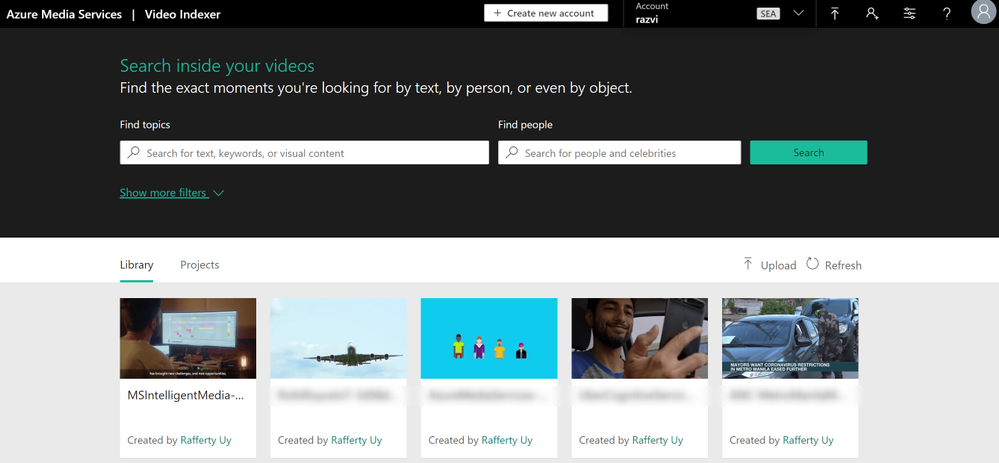
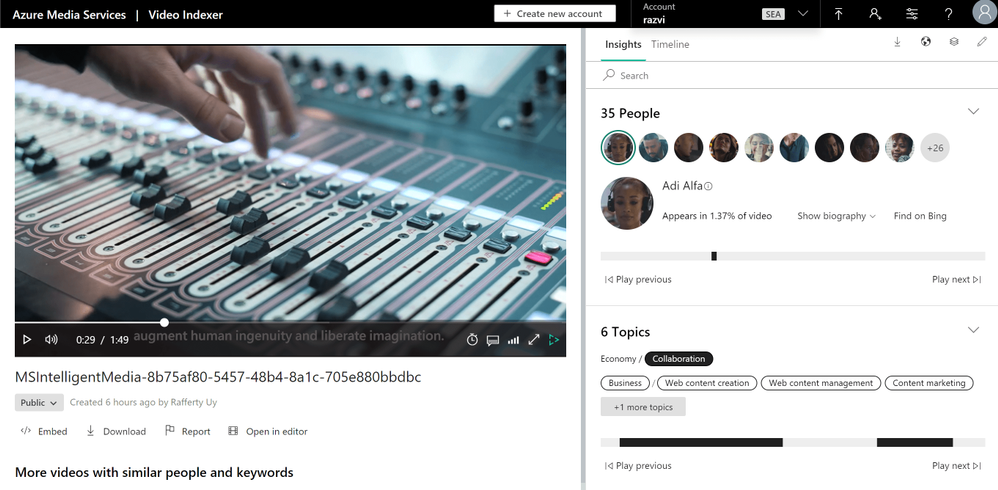
Publish Asset for Streaming
Publishing an asset in this article’s definition is about creating a streaming locator so that the asset is available for VOD.
The Logic App
This is a pretty simple logic app and could have been combined with the previous one. But there are many reasons for not publishing an asset immediately after transcoding such as:
- Checking the quality of the video,
- Adding AES or DRM encryption policies,
- Adding closed captions,
- and more
So to disconnect, I used an HTTP POST request to trigger this logic app, with the following JSON content-type body.
{
"properties": {
"alternative-media-id": {
"type": "string"
},
"assetName": {
"type": "string"
},
"contentKeyPolicyName": {
"type": "string"
},
"endDateTime": {
"type": "string"
},
"startDateTime": {
"type": "string"
},
"streamingEndpointName": {
"type": "string"
},
"streamingLocatorId": {
"type": "string"
},
"streamingPolicyName": {
"type": "string"
}
},
"type": "object"
}
A simple request body will look like this:
{
"assetName": "MSIntelligentMedia-679c31f7-6cb1-47ad-bf92-080e19830c64",
"streamingPolicyName": "Predefined_ClearStreamingOnly"
}
With this HTTP request, it will be as simple as calling the PublishAsset and GetAssetUrls Azure Functions.
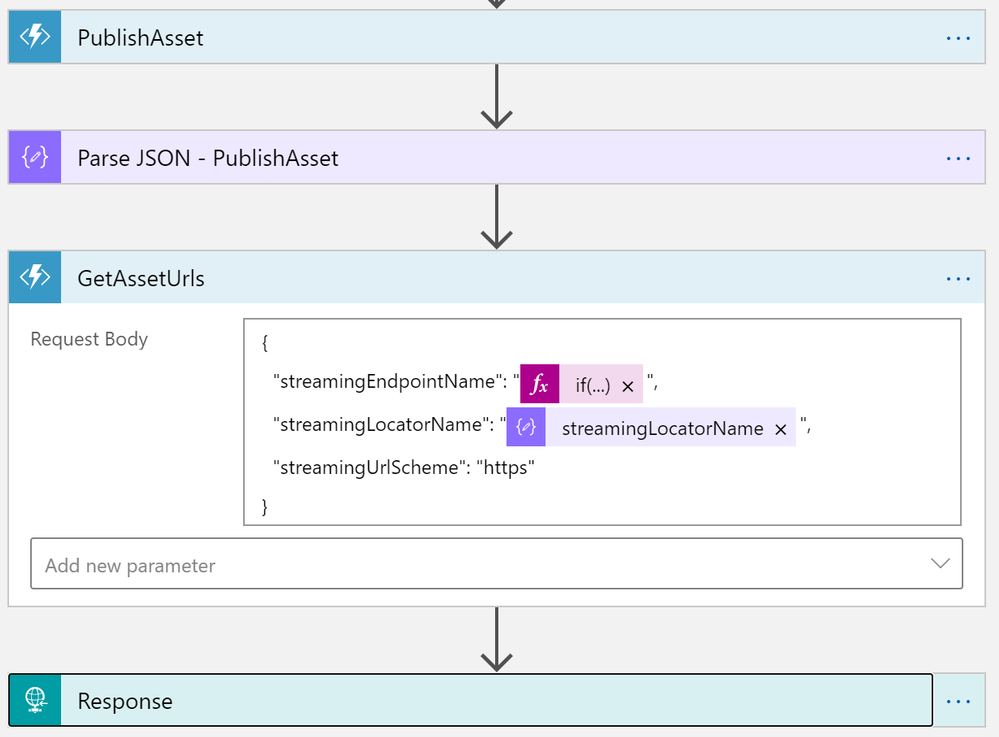
Output
This HTTP request will give me the following output.
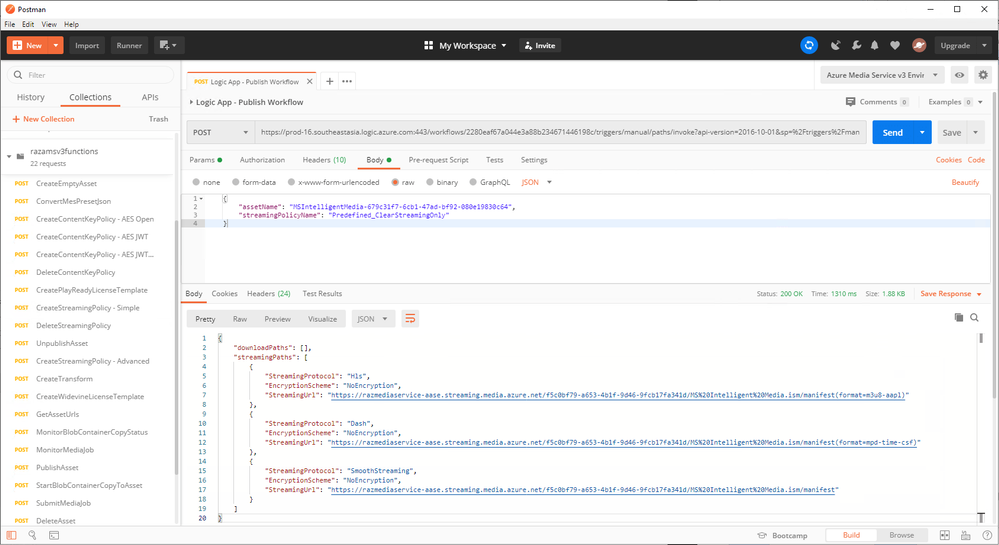
Conclusion
In this post, we learned how to create an Upload-and-Publish workflow using Azure Logic Apps and Azure Functions. Azure Functions for calling the Azure Media Service APIs and Logic Apps to orchestrate the workflow. This post also shows that Azure Logic Apps is not just a low-code/no-code tool. It is fully capable of integrating with your custom code.
GitHub Repository: https://github.com/raffertyuy/media-services-v3-dotnet-core-functions-integration
by Scott Muniz | Aug 18, 2020 | Azure, Microsoft, Technology, Uncategorized
This article is contributed. See the original author and article here.
The August update includes the following new features, bugs fixes, changes, and improvements.
Preview
We are previewing Java Message Service (JMS) 2.0 for Azure Service Bus premium tier. The JMS 2.0 APIs empower customers to seamlessly lift and shift their Spring workloads to Azure, while helping them modernize their application stack with best in class enterprise messaging in the cloud. As an example, the Azure service bus starter will now auto create queues and topics, at runtime over AMQP, if they don’t already exist.
New
- Connection to multiple Key Vault from a single application configuration file
- Support case sensitive keys in Key Vault
- Key Vault Spring Boot Actuator
See our key vault documentation on how to leverage these new features.
Improved
We revamped the refresh logic in Azure Key Vault to better adhere to refresh intervals defined in application configuration properties, and avoid unnecessary updates.
Fixed
We upgraded the Spring Boot dependency to 2.3.2, which addresses many CVEs and cleaned up all warnings at build time.
Changed
We have adopted the mono-repo approach. Azure Spring Starters have migrated from the 4 previous locations under https://github.com/microsoft/ to https://github.com/Azure/azure-sdk-for-java/tree/master/sdk. Developers can now easily find all Azure Spring starters under this single repository under the Azure SDK for java folder. Furthermore, this change allows us to bring more timely updates from Azure service SDKs and run more regression tests for quality control and preserve compatibility integrity.
Lastly the following starters are deprecated
- azure-servicebus-spring-boot-starter
- azure-mediaservices-spring-boot-starter
- azure-storage-spring-boot-starter
Recent Comments