by Contributed | May 13, 2023 | Technology
This article is contributed. See the original author and article here.
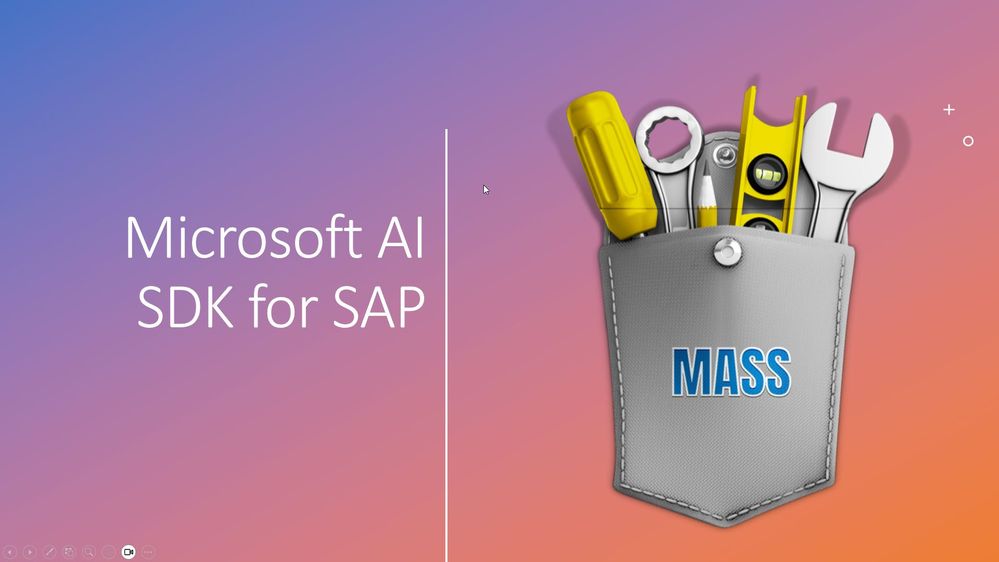
We are excited to announce the launch of Microsoft AI SDK for SAP ABAP. This software development kit (SDK) is designed to provide SAP ABAP developers with the tools they need to create intelligent enterprise applications using Artificial Intelligence (AI) technologies.
Engineered with a deep understanding of developers’ needs, the Microsoft AI SDK for SAP ABAP presents an intuitive interface that effortlessly brings AI capabilities to your ABAP applications. This toolkit offers an exciting avenue to tap into the power of Azure OpenAI. And this is just the beginning — our commitment to progress promises the inclusion of even more AI engines in future versions.
Azure OpenAI, the crown jewel of Microsoft Azure’s offerings, is a powerhouse of AI services and tools. It is your passport to harnessing machine learning algorithms, leveraging advanced natural language processing tools, and exploring versatile cognitive services. Its vast suite of tools paves the way for the creation of intelligent applications that excel in pattern detection, natural language processing, and data-driven predictions. Azure OpenAI grants you access to an array of pre-built AI models and algorithms, along with custom model training and deployment tools, all under the umbrella of stringent security, compliance, and data privacy standards.
With the AI SDK for SAP ABAP and Azure OpenAI integration with SAP, developers are on the brink of a new frontier. Now you have the power to craft innovative applications that can revolutionize the enterprise landscape by automating mundane tasks, bolstering smarter business decisions, and providing a more personalized customer experience. It’s more than a development kit — it’s your passport to an exciting future of technological evolution for enterprises running on the SAP platform.
Features:
The Microsoft AI SDK for SAP ABAP v1.0 is not just a toolset, it’s an innovation accelerator, an efficiency propellant. Designed for ABAP developers, it supercharges their workflows, slashing the time taken to integrate cutting-edge AI capabilities. With its streamlined integration process and ABAP-ready data types, developers can fast-track their tasks and concentrate on their real mission – crafting intelligent, transformative applications. This is no ordinary toolkit; it’s your express lane to the future of enterprise software development.
- Extensive Capabilities: It provides a comprehensive suite of functionalities, including Models, Deployment, Files, Fine-Tuning, and Completion (GPT3), along with Chat Completion (GPT4) capabilities.
- ABAP-Ready Data Types: We’ve simplified the integration process for ABAP developers by offering ABAP-ready data types. This feature substantially lowers the entry barriers, enabling developers to leverage the SDK with ease.
- Azure OpenAI Support: The SDK is fully compatible with Azure OpenAI, ensuring seamless integration and performance.
- Enterprise Control: To safeguard sensitive data, we’ve incorporated a robust enterprise control mechanism, offering three levels of control granularity. Enterprises can effectively manage SDK usage by implementing policies to permit or block specific functionalities. For instance, an organization could use authorizations to designate a user group capable of performing setup operations (Deployment, Files, and Fine-Tuning), while enabling all users to utilize the Completions functionality.
- Flexible Authentication: The SDK supports authentication using either Azure OpenAI Keys or Azure Active Directory (AAD), providing users with a secure and flexible approach to authentication.
In this age of relentless technological progress, AI is undeniably the cornerstone of enterprise software development’s future. The Microsoft AI SDK for SAP ABAP is a dynamic and transformative tool, purpose-built for SAP professionals. It’s not just a toolkit; it’s a supercharger for your innovative instincts, enabling you to build intelligent, data-centric applications. Our aim is to help businesses stay nimble and competitive in a marketplace where the pace of innovation is breakneck.
The launch of the Microsoft AI SDK for SAP ABAP is a leap into the future. It encapsulates our commitment to fostering the symbiotic relationship between technology and business, nurturing an environment where the opportunities for innovation are limitless. As we unfurl this state-of-the-art tool, we can’t wait to see the inventive applications that you, the talented developers working within the SAP ecosystem, will craft. The potential is staggering, poised to redefine how businesses operate and flourish.
And our commitment doesn’t stop at providing you with the tools. We pledge unwavering support on your journey of discovery and innovation with the Microsoft AI SDK for SAP ABAP. We’re with you every step of the way — to guide, support, and celebrate as you traverse this transformative technological landscape. Let’s stride boldly together into this new era of intelligent, data-driven enterprise solutions. The future is here, and it’s brighter than ever.
Best Regards,
Gopal Nair – Principal Software Engineer, Microsoft, – Author
Amit Lal – Principal Technical Specialist, Microsoft – Contributor
Join us and share your feedback: Azure Feedback
#MicrosoftAISDK #AISDKforSAPABAP #EnterpriseGPT #GPT4 #AzureOpenAI #SAPonAzure #SAPABAP
Disclaimer: The announcement of the Microsoft AI SDK for SAP ABAP is intended for informational purposes only. Microsoft reserves the right to make adjustments or changes to the product, its features, availability, and pricing at any time without prior notice. This blog does not constitute a legally binding offer or guarantee of specific functionalities or performance characteristics. Please refer to the official product documentation and agreements for detailed information about the product and its use. Microsoft is deeply committed to the responsible use of AI technologies. It is recommended to review and comply with all applicable laws, regulations, and organizational policies to ensure the responsible and ethical use of AI.
by Contributed | May 13, 2023 | Technology
This article is contributed. See the original author and article here.
For Azure log alert notification action using logic app, we have read numerous articles. But I notice that most of them are either very brief or don’t go into great detail about all the nuances, tips, or tricks. I therefore wanted to write one with as much detail as I could and some fresh additional strategies. I hope this aids in developing the logic and putting it into practise.
So let’s get going. We already know that creating the Alert rule and choosing the logic app as the action are necessary. Additionally, the Logic app’s alert notification trigger for when an HTTP request is received. So let’s construct one.
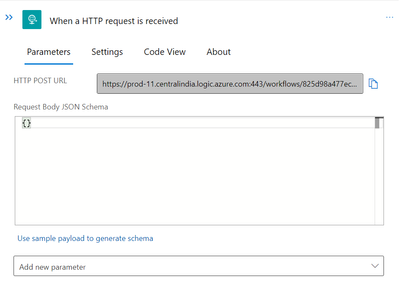
We can use the below sample schema for the above trigger task
{
“type”: “object”,
“properties”: {
“schemaId”: {
“type”: “string”
},
“data”: {
“type”: “object”,
“properties”: {
“essentials”: {
“type”: “object”,
“properties”: {
“alertId”: {
“type”: “string”
},
“alertRule”: {
“type”: “string”
},
“severity”: {
“type”: “string”
},
“signalType”: {
“type”: “string”
},
“monitorCondition”: {
“type”: “string”
},
“monitoringService”: {
“type”: “string”
},
“alertTargetIDs”: {
“type”: “array”,
“items”: {
“type”: “string”
}
},
“configurationItems”: {
“type”: “array”,
“items”: {
“type”: “string”
}
},
“originAlertId”: {
“type”: “string”
},
“firedDateTime”: {
“type”: “string”
},
“description”: {
“type”: “string”
},
“essentialsVersion”: {
“type”: “string”
},
“alertContextVersion”: {
“type”: “string”
}
}
},
“alertContext”: {
“type”: “object”,
“properties”: {
“properties”: {},
“conditionType”: {
“type”: “string”
},
“condition”: {
“type”: “object”,
“properties”: {
“windowSize”: {
“type”: “string”
},
“allOf”: {
“type”: “array”,
“items”: {
“type”: “object”,
“properties”: {
“searchQuery”: {
“type”: “string”
},
“metricMeasureColumn”: {},
“targetResourceTypes”: {
“type”: “string”
},
“operator”: {
“type”: “string”
},
“threshold”: {
“type”: “string”
},
“timeAggregation”: {
“type”: “string”
},
“dimensions”: {
“type”: “array”
},
“metricValue”: {
“type”: “integer”
},
“failingPeriods”: {
“type”: “object”,
“properties”: {
“numberOfEvaluationPeriods”: {
“type”: “integer”
},
“minFailingPeriodsToAlert”: {
“type”: “integer”
}
}
},
“linkToSearchResultsUI”: {
“type”: “string”
},
“linkToFilteredSearchResultsUI”: {
“type”: “string”
},
“linkToSearchResultsAPI”: {
“type”: “string”
},
“linkToFilteredSearchResultsAPI”: {
“type”: “string”
}
},
“required”: [
“searchQuery”,
“metricMeasureColumn”,
“targetResourceTypes”,
“operator”,
“threshold”,
“timeAggregation”,
“dimensions”,
“metricValue”,
“failingPeriods”,
“linkToSearchResultsUI”,
“linkToFilteredSearchResultsUI”,
“linkToSearchResultsAPI”,
“linkToFilteredSearchResultsAPI”
]
}
},
“windowStartTime”: {
“type”: “string”
},
“windowEndTime”: {
“type”: “string”
}
}
}
}
},
“customProperties”: {}
}
}
}
}
However, as can be seen, the output above is insufficient to provide a thorough error message for the notification. In order to receive the message, we must perform additional tasks.
The same query that was used in the Alert rule can be run again with additional filtering options to produce the error code and message shown below.
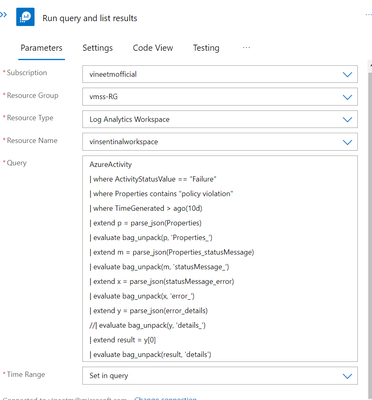
The aforementioned query serves as an example of how to extract the error message using multiple iterations from the Properties field.
Now, initialise the variables as shown below.
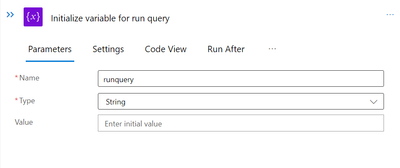
By choosing type as “String,” we must create 4 “Initialise Variable” tasks for “Runquery,” “Owner,” “HTMLtable,” and “Authorise.”
Also keep in mind that the List query result may contain multiple logs. Therefore, we will use a foreach loop to go through each error log one at a time and send notifications for each one. Let’s create the following foreach task to accomplish that.
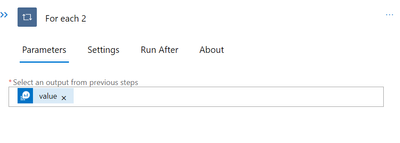
The result of the “Run query and list result” task is the value.
The next step is to retrieve the current Log from a variable we previously initialised. Let’s now set the value for that variable using the current item from the Foreach task.
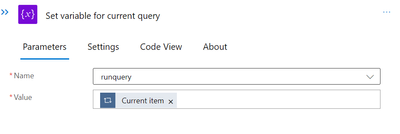
The value is the output of the “Run query and list result” task.
The next step is to get the most recent Log from a variable that was initialised earlier. Using the current item from the Foreach task, let’s now set the value for that variable.
So that we can obtain the field values in subsequent tasks, parse this variable into JSON. You can simply run the Logicapp to obtain the output for the aforementioned variable in order to obtain the schema for this task. Next, duplicate that output and paste it into the sample payload link for the task below.
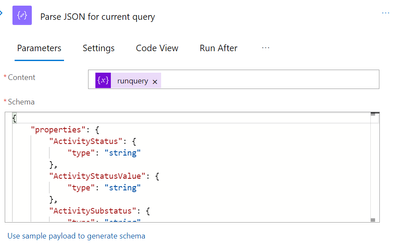
Our actual strategy is to e-mail or notify each error log. In this instance, the owner of the subscription will receive the email containing the reported error or violation.
We must make sure the logs are captured, which is done in the Alert rule window itself, because the query will be run once more after the alert. So let’s add a requirement to only gather those logs.
In order to ensure that the TimeGenerated field is between the Alert rule (trigger task), we will create a Condition task and choose it from the aforementioned “Parse JSON task.” Windows commencement and termination
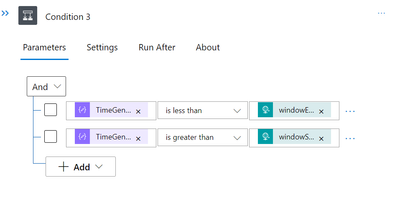
Now If it is accurate, we can move on to obtaining the owner user or users’ information. However, let’s use HTTP action for API GET call if you have numerous subscriptions and want to display Subscription Name in your Notification as well. Use the API link as shown below and the SubscriptionID from the Current query Parse JSON task.
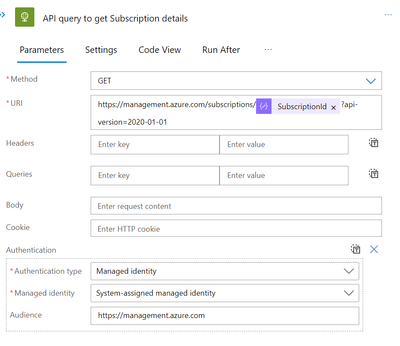
You can choose Managed Identity (of Logicapp) as your authentication type. You can choose Identity from the main menu list in the Logicapp, enable Managed Identity, and grant Reader permission for each subscription before setting this task.
Run the logicapp now to obtain the results of the aforementioned API request. To have the attributes of a subscription, copy the output and paste it into the sample payload for the subsequent Parse JSON task.
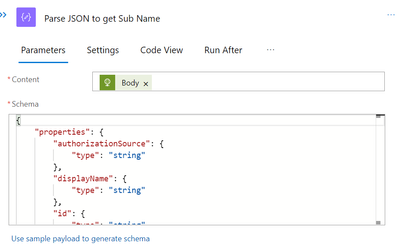
The Owners must now be filtered by the Subscription Users. Let’s make another HTTP action for the API GET task to accomplish that.
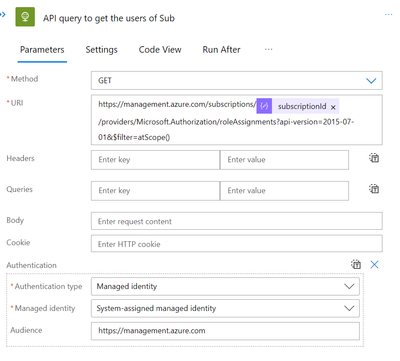
Let’s run the logicapp once more to obtain the results of this API task, then copy and paste them into the sample payload for the subsequent Parse JSON task in order to obtain the schema. Make sure the Content you choose is the API task’s body from above.
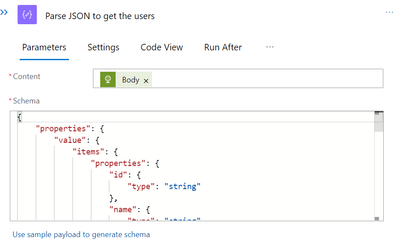
We currently have access to every subscription for the current log. To send the notification, however, we only need the Owner user. Therefore, we must use the Foreach task once more to filter each user and find the Owner user. The output of the previous parse JSON task serves as the Value for this.
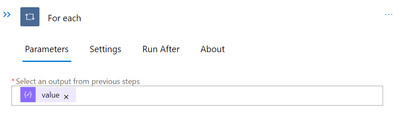
Let’s now enter the details of the current user into a variable. Keep in mind that we previously initialised the variable “owner.” To set the value for it, create a Set Variable task now. Make sure the value represents the result of the previous foreach task.
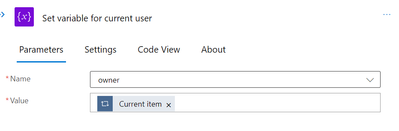
To get the attribute values of the current user for later use, we must now parse the Variable into JSON.
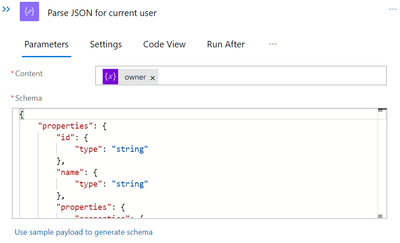
To obtain the output of the aforementioned variable and obtain the schema, we must once again run the logicapp and copy/paste the results to the sample payload link above.
To identify the Owner user, we must now obtain the Owner’s Role AssignmentID (which is common in Azure). To obtain the Role Assignment ID, go to your subscription’s IAM (Access Control), click Role Assignments, then select any Owner and the JSON tab. However, you can also use PowerShell/CLI. Alternately, you can use the logicapp to validate the owner’s role assignment ID after receiving the output of the aforementioned “Parse JSON for Users” task. For future use, copy that.
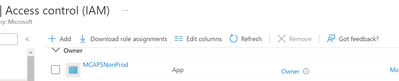
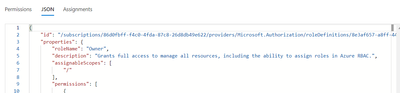
The ID guid value can also be copied from the ID value.
To select only the Owner user for subsequent tasks, we must now create a Condition task to filter the users. The ID field from the task “Parse JSON for current user” should be used as the condition field.
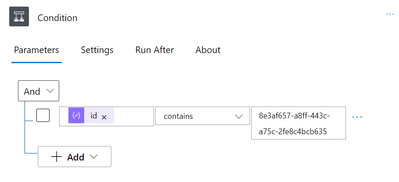
The most crucial thing to keep in mind right now is that we must run a Graph API query in order to obtain user attributes like email and UPN, etc. For obtaining those attributes, the results of the current API queries are insufficient. But we need the following permission in the AAD in order to access those users’ attributes. The SPN (app registration) must be created, the following API permissions must be provided, and admin consent must be granted.
Permission
|
Type
|
Directory.AccessAsUser.All
|
Delegated
|
Directory.ReadWrite.All
|
Delegated
|
Directory.ReadWrite.All
|
Application
|
Group.ReadWrite.All
|
Delegated
|
Group.ReadWrite.All
|
Application
|
User.Read
|
Delegated
|
User.Read.All
|
Delegated
|
User.Read.All
|
Application
|
User.ReadWrite.All
|
Delegated
|
User.ReadWrite.All
|
Application
|
Additionally, duplicate the App ID and Tenant ID, make a secret, and copy the Secret key for the subsequent task.
To run a Graph API query, we must now execute the following HTTP action for API task. To obtain information about the current user, use the ‘Parse Json for current user’s principalID’ command.
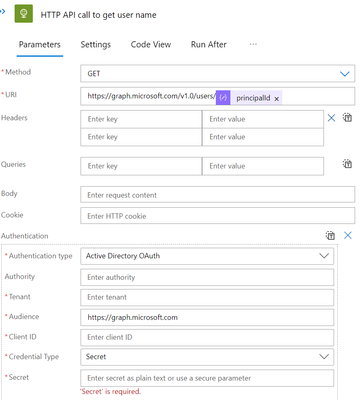
Choose the Authentication parameter and enter the SPN-copied Tenant ID, App ID (Client ID), and Secret.
For the output from the aforementioned API, create a new “Parse JSON” task. To obtain the output of the aforementioned task’s sample payload to paste into the parse json task’s payload to obtain the schema, we can run the logicapp once more.
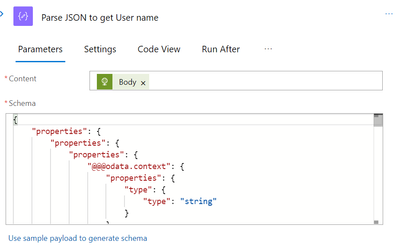
We should now have a good format for the notification to appear in the email. We’ll use an HTML table for that, filled with information from the query above (such as the error code, error message, severity, and subname). Although you are free to use your own format, you can use the sample provided by this github link (attached below) as a guide. You must choose the HTML table (the initialise variable we created earlier) and use the ‘Set Variable’ task to paste the value from the example HTML code I’ve attached below.
<>
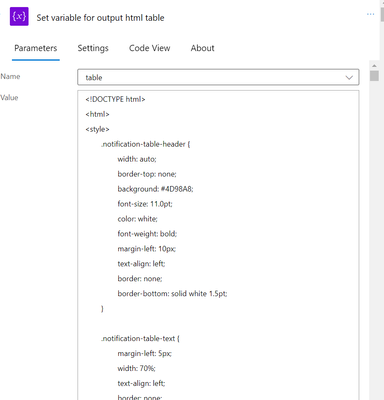
Update the fields/values as indicated below in the code at the appropriate lines/locations.
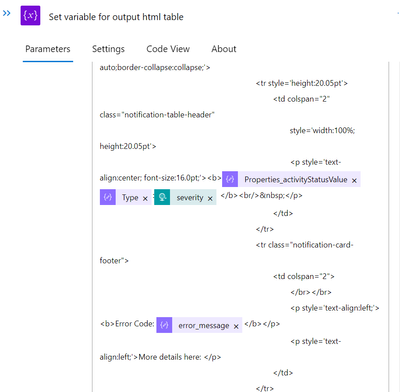
After that, a task called Send email V2 can be created in Outlook 365 to send the notification.
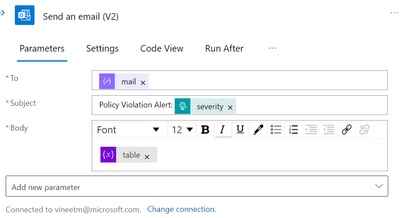
You will receive an email as below.
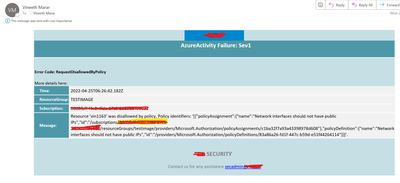
Before we go any further, make sure your Alert rule in Azure Monitor has been created and the aforementioned logicapp has been selected as the action. Make sure the error/administration diagnostic logs are enabled to send to the Log analytics workspace for all subscriptions. If you want to set up separate alert rules for “Error” and “Critical,” create them separately and choose the same logicapp as the action. Here is just a sample.
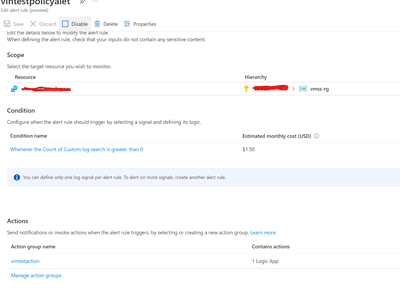
And the Condition query should be as below (you can modify as per your requirement)
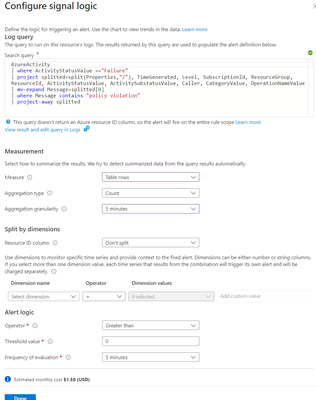
The evaluation of the log analytics workspace (activity logs) will be performed every 5 minutes, and if any policy violation errors are discovered, an alert will be sent. The Logic app will be activated as soon as the Alert is fired, and the Owner of the resource subscription will receive a notification email in the format shown above with all necessary information.
Hope you had a great reading and happy learning.
by Contributed | May 12, 2023 | Technology
This article is contributed. See the original author and article here.
OpenFOAM (Open Field Operation and Manipulation) is an open-source computational fluid dynamics (CFD) software package. It provides a comprehensive set of tools for simulating and analyzing complex fluid flow and heat transfer phenomena. It is widely used in academia and industry for a range of applications, such as aerodynamics, hydrodynamics, chemical engineering, environmental simulations, and more.
Azure offers services like Azure Batch and Azure CycleCloud that can help individuals or organizations run OpenFOAM simulations effectively and efficiently. In both scenarios, these services allow users to create and manage clusters of VMs, enabling parallel processing and scaling of OpenFOAM simulations. While CycleCloud provides a similar experience to on-premises thanks to its support to common schedulers like OpenPBS or SLURM; Azure Batch provides a cloud native resource scheduler that simplifies the configuration, maintenance and support of your required infrastructure.
This article covers a step-by-step guide on a minimal Azure Batch setup to run OpenFOAM simulations. Further analysis should be performed to identify the right sizing both in terms of compute and storage. A previous article on How to identify the recommended VM for your HPC workloads could be helpful.
Step 1: Provisioning required infrastructure
To get started, create a new Azure Batch account. At this point a pool, job or task is not required. In our scenario, the pool allocation method would be configure as “User Subscription” and public network access configured to “All Networks”.
A shared storage across all nodes would be also required to share the input model and store the outputs. In this guide, an Azure Files NFS share would be used. Alternatives like Azure NetApp Files or Azure Managed Lustre could also be an option base on your scalability and performance needs.
Step 2: Customizing the virtual machine image
OpenFOAM provides pre-compiled binaries packaged for Ubuntu that can be installed through its oficial APT repositories. If Ubuntu is your distribution of choice, you can follow the oficial documentation on how to install it, using a pool’s start task is a good approach to do it. As an alternative, you can create a custom image with everything already pre-configured.
This article would cover the second option using CentOS 7.9 as base image to show the end-to-end configuration and compilation of the software from source code. To simplify the process, it would rely on the available HPC images that provide the required pre-requisites already installed. The reference URN for those images is: OpenLogic:CentOS-HPC:s7_9-gen2:latest. The SKU of the VM we would use both to create the custom image and run the simulations is a HBv3.
Start the configuration creating a new VM. After the VM is up and running, execute the following script to download and compile OpenFOAM source code.
## Downloading OpenFoam
sudo mkdir /openfoam
sudo chmod 777 /openfoam
cd /openfoam
wget https://dl.openfoam.com/source/v2212/OpenFOAM-v2212.tgz
wget https://dl.openfoam.com/source/v2212/ThirdParty-v2212.tgz
tar -xf OpenFOAM-v2212.tgz
tar -xf ThirdParty-v2212.tgz
module load mpi/openmpi
module load gcc-9.2.0
## OpenFoam 10 requires cmake 3. CentOS 7.9 cames with a previous version.
sudo yum install epel-release.noarch -y
sudo yum install cmake3 -y
sudo yum remove cmake -y
sudo ln -s /usr/bin/cmake3 /usr/bin/cmake
source OpenFOAM-v2212/etc/bashrc
foamSystemCheck
cd OpenFOAM-v2212/
./Allwmake -j -s -q -l
The last command compiles with all cores (-j), reduced output (-s, -silent), with queuing (-q, -queue) and logs (-l, -log) the output to a file for later inspection. After the initial compilation, review the output log or re-run the last command to make sure that everything was compiled without errors. Output is so verbose that errors could be missed in a quick review of the logs.
It would take a while before the compilation process finishes. After that, you can delete the installers and any other folder not required in your scenario and capture the image into a Shared Image Gallery.
Step 3. Batch pool configuration
Add a new pool to your previously created Azure Batch account. You can create a new pool using the standard wizard (Add) and fulfilling the required fields with the values mentioned in the following JSON, or you can copy and paste this file into the Add (JSON editor).
Make sure you customize the properties between .
{
"properties": {
"vmSize": "STANDARD_HB120rs_V3",
"interNodeCommunication": "Enabled",
"taskSlotsPerNode": 1,
"taskSchedulingPolicy": {
"nodeFillType": "Pack"
},
"deploymentConfiguration": {
"virtualMachineConfiguration": {
"imageReference": {
"id": ""
},
"nodeAgentSkuId": "batch.node.centos 7",
"nodePlacementConfiguration": {
"policy": "Regional"
}
}
},
"mountConfiguration": [
{
"nfsMountConfiguration": {
"source": "",
"relativeMountPath": "data",
"mountOptions": "-o vers=4,minorversion=1,sec=sys"
}
}
],
"networkConfiguration": {
"subnetId": "",
"publicIPAddressConfiguration": {
"provision": "BatchManaged"
}
},
"scaleSettings": {
"fixedScale": {
"targetDedicatedNodes": 0,
"targetLowPriorityNodes": 0,
"resizeTimeout": "PT15M"
}
},
"targetNodeCommunicationMode": "Simplified"
}
}
Wait till the pool is created and the nodes are available to accept new tasks. Your pool view should look similar to the following image.
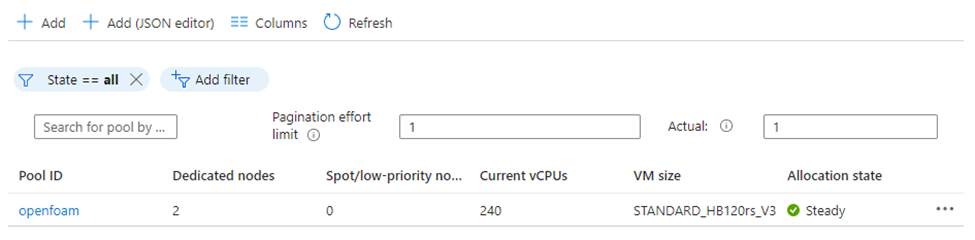
Step 4. Batch Job Configuration
Once the pool allocation state value is “Ready”, continue with the next step: create a new Job. Default configuration is enough in this case. In our case, the job is called “flange” because we would use the flange example from OpenFOAM tutorials.

Step 5. Task Pool Configuration
Once the job state value changes to “Active”, it is ready to admit new tasks. You can create a new task using the standard wizard (Add) and fulfilling the required fields with the values mentioned in the following JSON, or you can copy and paste this file into the Add (JSON editor).
Make sure you customize the properties between .
{
"id": "",
"commandLine": "/bin/bash -c '$AZ_BATCH_NODE_MOUNTS_DIR/data/init.sh'",
"resourceFiles": [],
"environmentSettings": [],
"userIdentity": {
"autoUser": {
"scope": "pool",
"elevationLevel": "nonadmin"
}
},
"multiInstanceSettings": {
"numberOfInstances": 2,
"coordinationCommandLine": "echo "Coordination completed!"",
"commonResourceFiles": []
}
}
Task commandline parameter is configured to execute a Bash script stored into the Azure Files that Batch is mounting automatically into the ‘$AZ_BATCH_NODE_MOUNTS_DIR/data’ folder. You need to copy first the following scripts and the flange example mentioned above into a folder called flange inside that directory.
Command Line Task Script
This script would configure the environment variables and pre-process the input files before launching the mpirun command to execute the solver in parallel across all the available nodes. In this case, 2 nodes with 240 cores.
#! /bin/bash
source /etc/profile.d/modules.sh
module load mpi/openmpi
# Azure Files is mounted automatically in this directory based on the pool configuration
DATA_DIR="$AZ_BATCH_NODE_MOUNTS_DIR/data"
# OpenFoam was installed on this folder
OF_DIR="/openfoam/OpenFOAM-v2212"
# A new folder is created per execution and the input data copied there.
mkdir -p "$DATA_DIR/flange"
unzip -o "$DATA_DIR/flange.zip" -d "$DATA_DIR/$AZ_BATCH_TASK_ID"
# Configures OpenFoam environment
source "$OF_DIR/etc/bashrc"
source "$OF_DIR/bin/tools/RunFunctions"
# Preprocessing of the files
cd "$DATA_DIR/$AZ_BATCH_JOB_ID-flange"
runApplication ansysToFoam "$OF_DIR/tutorials/resources/geometry/flange.ans" -scale 0.001
runApplication decomposePar
# Configure the host file
echo $AZ_BATCH_HOST_LIST | tr "," "n" > hostfile
sed -i 's/$/ slots=120/g' hostfile
# Launching the secondarr script to perform the parallel computation.
mpirun -np 240 --hostfile hostfile "$DATA_DIR/run.sh" > solver.log
Mpirun Processing Script
This script would launch the task in all the nodes available. It is required to configure the environment variables and folders the solver would need to access. If this script is not executed and the solver is invoked directly on the mpirun command, only the primary task node would have the right configuration applied and the rest of the nodes would fail with file not found errors.
#! /bin/bash
source /etc/profile.d/modules.sh
module load gcc-9.2.0
module load mpi/opennmpi
DATA_DIR="$AZ_BATCH_NODE_MOUNTS_DIR/data"
OF_DIR="/openfoam/OpenFOAM-v2212"
source "$OF_DIR/etc/bashrc"
source "$OF_DIR/bin/tools/RunFunctions"
# Execute the code across the nodes.
laplacianFoam -parallel > solver.log
Step 6. Checking the results
Mpirun output is redirected to a file called solver.log in the directory where the model is stored inside the Azure Files file share. Checking the first lines of the log, it’s possible to validate that the execution has properly started and it’s running on top of two HBv3 with 240 processes.
/*---------------------------------------------------------------------------*
| ========= | |
| / F ield | OpenFOAM: The Open Source CFD Toolbox |
| / O peration | Version: 2212 |
| / A nd | Website: www.openfoam.com |
| / M anipulation | |
*---------------------------------------------------------------------------*/
Build : _66908158ae-20221220 OPENFOAM=2212 version=v2212
Arch : "LSB;label=32;scalar=64"
Exec : laplacianFoam -parallel
Date : May 04 2023
Time : 15:01:56
Host : 964d5ce08c1d4a7b980b127ca57290ab000000
PID : 67742
I/O : uncollated
Case : /mnt/resource/batch/tasks/fsmounts/data/flange
nProcs : 240
Hosts :
(
(964d5ce08c1d4a7b980b127ca57290ab000000 120)
(964d5ce08c1d4a7b980b127ca57290ab000001 120)
)
Conclusion
By leveraging Azure Batch’s scalability and flexible infrastructure, you can run OpenFOAM simulations at scale, achieving faster time-to-results and increased productivity. This guide demonstrated the process of configuring Azure Batch, customizing the CentOS 7.9 image, installing dependencies, compiling OpenFOAM, and running simulations efficiently on Azure Batch. With Azure’s powerful capabilities, researchers and engineers can unleash the full potential of OpenFOAM in the cloud.
by Contributed | May 11, 2023 | Technology
This article is contributed. See the original author and article here.
Spear phishing campaign is a type of attack where phishing emails are tailored to specific organization, organization’s department, or even specific person. Spear phishing is a targeted attack by its definition and rely on preliminary reconnaissance, so attackers are ready to spend more time and resources to achieve their targets. In this blog post, we will discuss steps that can be taken to respond to such a malicious mailing campaign using Microsoft 365 Defender.
What makes phishing “spear”
Some of the attributes of such attacks are:
- Using local language for subject, body, and sender’s name to make it harder for users to identify email as phishing.
- Email topics correspond to the recipient’s responsibilities in the organization, e.g., sending invoices and expense reports to the finance department.
- Using real compromised mail accounts for sending phishing emails to successfully pass email domain authentication (SPF, DKIM, DMARC).
- Using large number of distributed mail addresses to avoid bulk mail detections.
- Using various methods to make it difficult for automated scanners to reach malicious content, such as encrypted ZIP-archives or using CAPTCHA on phishing websites.
- Using polymorphic malware with varying attachment names to complicate detection and blocking.
In addition to reasons listed above, misconfigured mail filtering or transport rules can also lead to the situation where malicious emails are hitting user’s inboxes and some of them can eventually be executed.
Understand the scope of attack
After receiving first user reports or endpoint alerts, we need to understand the scope of attack to provide adequate response. To better understand the scope, we need to try to answer the following questions:
- How many users are affected? Is there anything common between those users?
- Is there anything shared across already identified malicious emails, e.g. mail subject, sender address, attachment names, sender domain, sender mail server IP address?
- Are there similar emails delivered to other users within the same timeframe?
Basic hunting will need to be done at this point, starting with information we have on reported malicious email, luckily Microsoft 365 Defender provides extensive tools to do that. For those who prefer interactive UI, Threat Explorer is an ideal place to start.
Figure 1: Threat Explorer user interface
Using filter at the top, identify reported email and try to locate similar emails sent to your organization, with the same parameters, such as links, sender addresses/domains or attachments.
Figure 2: Sample mail filter query in Threat Explorer
For even more flexibility, Advanced Hunting feature can be used to search for similar emails in the environment. There are five tables in Advanced Hunting schema that contain Email-related data:
- EmailEvents – contains general information about events involving the processing of emails.
- EmailAttachmentInfo – contains information about email attachments.
- EmailUrlInfo – contains information about URLs on emails and attachments.
- EmailPostDeliveryEvents – contains information about post-delivery actions taken on email messages.
- UrlClickEvents – contains information about Safe Links clicks from email messages
For our purposes we will be interested in the first three tables and can start with simple queries such as the one below:
EmailAttachmentInfo
| where Timestamp > ago(4h)
| where FileType == "zip"
| where SenderFromAddress has_any (".br", ".ru", ".jp")
This sample query will show all emails with ZIP attachments received from the same list of TLDs as identified malicious email and associated with countries where your organization is not operating. In a similar way we can hunt for any other attributes associated with malicious emails.
Check mail delivery and mail filtering settings
Once we have some understanding of how attack looks like, we need to ensure that the reason for these emails being delivered to user inboxes is not misconfiguration in mail filtering settings.
Check custom delivery rules
For every mail delivered to your organization, Defender for Office 365 provides delivery details, including raw message headers. Right from the previous section, whether you used Threat Explorer or Advanced Hunting, by selecting an email item and clicking Open email entity button, you can pivot to email entity page to view all the message delivery details, including any potential delivery overrides, such as safe lists or Exchange transport rules.
Figure 3: Sample email with delivery override by user’s safe senders list
It might be the case that email was properly detected as suspicious but was still delivered to mailbox due to an override, like on screenshot above where sender is on user’s Safe Senders list, other delivery override types are:
- Allow entries for domains and email addresses (including spoofed senders) in the Tenant Allow/Block List.
- Mail flow rules (also known as transport rules).
- Outlook Safe Senders (the Safe Senders list that’s stored in each mailbox that affects only that mailbox).
- IP Allow List (connection filtering)
- Allowed sender lists or allowed domain lists (anti-spam policies)
If a delivery override has been identified, then it should be removed accordingly. Good news is that malware or high confidence phishing are always quarantined, regardless of the safe sender list option in use.
Check phishing mail header for on-prem environment
One more reason for malicious emails to be delivered to users’ inboxes can be found in hybrid Exchange deployments, where on-premises Exchange environment is not configured to handle phishing mail header appended by Exchange Online Protection.
Check threat policies settings
If there were no specific overrides identified it is always a good idea to double check mail filtering settings in your tenant, the easiest way to do that, is to use configuration analyzer that can be found in Email & Collaboration > Policies & Rules > Threat policies > Configuration analyzer:
Figure 4: Defender for Office 365 Configuration analyzer
Configuration analyzer will quickly help to identify any existing misconfigurations compared to recommended security baselines.
Make sure that Zero-hour auto purge is enabled
In Exchange Online mailboxes and in Microsoft Teams (currently in preview), zero-hour auto purge (ZAP) is a protection feature that retroactively detects and neutralizes malicious phishing, spam, or malware messages that have already been delivered to Exchange Online mailboxes or over Teams chat. Which exactly fits into the discussed scenario. This setting for email with malware can be found in Email & Collaboration > Policies & rules > Threat policies > Anti-malware. Similar setting for spam and phishing messages is located under Anti-spam policies. It is important to note that ZAP doesn’t work for on-premises Exchange mailboxes.
Figure 5: Zero-hour auto purge configuration setting in Anti-malware policy
Performing response steps
Once we have identified malicious emails and confirmed that all the mail filtering settings are in order, but emails are still coming through to users’ inboxes (see the introduction part of this article for reasons for such behavior), it is time for manual response steps:
Report false negatives to Microsoft
In Email & Collaboration > Explorer, actions can be performed on emails, including reporting emails to Microsoft for analysis:
Figure 6: Submit file to Microsoft for analysis using Threat Explorer
Actions can be performed on emails in bulk and during the submission process, corresponding sender addresses can also be added to Blocked senders list.
Alternatively, emails, specific URLs or attached files can be manually submitted through Actions & Submissions > Submissions section of the portal. Files can also be submitted using public website.
Figure 7: Submit file to Microsoft for analysis using Actions & submissions
Timely reporting is critical, the sooner researchers will get their hands on unique samples from your environment, and start their analysis, the sooner those malicious mails will be detected and blocked automatically.
Block malicious senders/files/URLs on your Exchange Online tenant
While you have an option to block senders, files and URLs during submission process, that can also be done without submitting using Email & Collaboration > Policies & rules > Threat policies > Tenant Allow/Block List, that UI also supports bulk operations and provides more flexibility.
Figure 8: Tenant Allow/Block Lists
The best way to obtain data for block lists is Advanced Hunting query, e.g. the following query can be used to return list of hashes:
EmailAttachmentInfo
| where Timestamp > ago(8h)
| where FileType == "zip"
| where FileName contains "invoice"
| distinct SHA256, FileName
Note: such a simple query might be too broad and include some legitimate attachments, make sure to adjust it further to get an accurate list and avoid false positive blockings.
Block malicious files/URLs/IP addresses on endpoints
Following defense-in-depth principle, even when malicious email slips through mail filters, we still have a good chance of detecting and blocking it on endpoints using Microsoft Defender for Endpoint. As an extra step, identified malicious attachments and URLs can be added as custom indicators to ensure their blocking on endpoints.
EmailUrlInfo
| where Timestamp > ago(4h)
| where Url contains "malicious.example"
| distinct Url
Results can be exported from Advanced Hunting and later on imported on Settings > Endpoints > Indicators page (Note: Network Protection needs to be enabled on devices to block URLs/IP addresses). The same can be done for malicious files using SHA256 hashes of attachments from EmailAttachmentInfo table.
Some other steps that can be taken to better prepare your organization for similar incident:
- Ensure that EDR Block Mode is enabled for machines where AV might be running in passive mode.
- Enable Attack Surface Reduction (ASR) rules to mitigate some of the risks associated with mail-based attacks on endpoints.
- Train your users to identify phishing mails with Attack simulation feature in Microsoft Defender for Office 365
Learn more
by Priyesh Wagh | May 11, 2023 | Dynamics 365, Microsoft, Technology
Here’s how you can reinstate an Exchange Online service if you see exception on your Mailbox by procuring an Exchange Online enabled license.
Brought to you by Dr. Ware, Microsoft Office 365 Silver Partner, Charleston SC.
Recent Comments