by Contributed | Apr 27, 2023 | Technology
This article is contributed. See the original author and article here.
We realize that a clear Windows client roadmap update helps consumers and organizations with planning their Windows release activities.
Today we’ll provide a brief update on the latest version of Windows 10, as well as share more on the time frame for the next Long-Term Servicing Channel (LTSC) release of Windows 11.
Windows 10 support lifecycle
As documented on the Windows 10 Enterprise and Education and Windows 10 Home and Pro lifecycle pages, Windows 10 will reach end of support on October 14, 2025. The current version, 22H2, will be the final version of Windows 10, and all editions will remain in support with monthly security update releases though that date. Existing LTSC releases will continue to receive updates beyond that date based on their specific lifecycles.
Recommendation
- We highly encourage you to transition to Windows 11 now as there won’t be any additional Windows 10 feature updates.
- If you and/or your organization must remain on Windows 10 for now, please update to Windows 10, version 22H2 to continue receiving monthly security update releases through October 14, 2025. See how you can quickly do this via a servicing enablement package in How to get the Windows 10 2022 Update.
The final end of support date for Windows 10 does not change with this announcement; these dates can be found on the Windows 10 Lifecycle page.
Windows 11 LTSC
It’s important for organizations to have adequate time to plan for adopting Windows 11. Today we’re announcing that the next Windows LTSC releases will be available in the second half of 2024:
- Windows 11 Enterprise LTSC
- Windows 11 IoT Enterprise LTSC
We’ll provide more details as we get closer to availability.
Recommendation
If you’re waiting for a Windows 11 LTSC release, you can begin planning and testing your applications and hardware on the current GA channel release, Windows 11, version 22H2. Check out App confidence: Optimize app validation with Test Base for more tips on how to test your applications.
Stay informed
In the future, we will add more information here and to the Windows release health page, which offers information about the General Availability Channel and LTSC under release information for appropriate versions.
The Windows release health page lists release information for different versions of Windows.
Continue the conversation. Find best practices. Bookmark the Windows Tech Community and follow us @MSWindowsITPro on Twitter. Looking for support? Visit Windows on Microsoft Q&A.
by Contributed | Apr 26, 2023 | Technology
This article is contributed. See the original author and article here.
With Azure Database for MySQL – Flexible Server, you can configure high availability with automatic failover within a region. The high availability solution is designed to ensure that committed data is never lost because of failures and that the database won’t be a single point of failure in your software architecture.
Note: For more information, see Azure Database for MySQL – Flexible Server – High Availability Concepts.
Within a region, there are three potential options to consider, as shown in the following table:
Option (Mode)
|
Committed SLA
|
Non-HA
|
99.9%
|
Same Zone HA
|
99.95%
|
Zone Redundant HA (ZHRA)*
|
99.99%
|
*ZRHA is only available in regions that support availability zones. For the latest list of Azure regions, in the Azure Database for MySQL documentation, see Azure regions.
In addition to the ‘in-region’ modes listed above, there’s also an option to design for protection of database services across Azure regions. One common pattern we’ve seen with several customers is the need for maximum in-region availability along with a cross region disaster recovery capability. This manifests itself as ZRHA in the primary region and a Read Replica in another region, preferably the paired region, as illustrated in the following diagram:
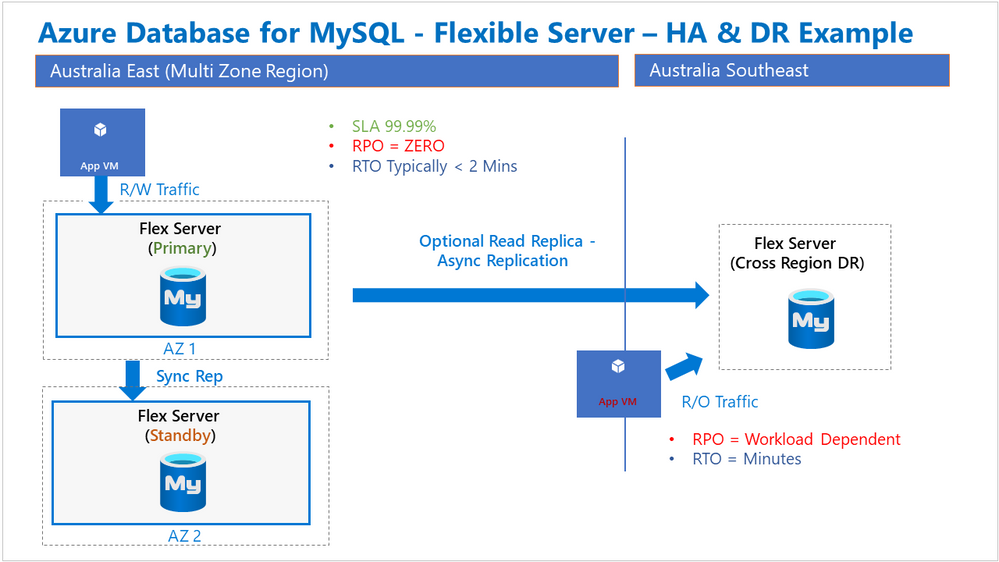
With ZRHA, failover between the Primary and Standby servers is automatically managed by the Azure platform, and importantly, the service endpoint name does not change. On the other hand, the manual process associated with a regional failover does introduce a change to the service endpoint name. Some customers have expressed an interest in being able to perform a regional failover without later having to update the associated application connection strings.
In this post, I’ll explain how to address this requirement and provide a regional failover that requires no application connection string changes.
For our purposes, we’ll use the following simplified architecture diagram as a starting point:
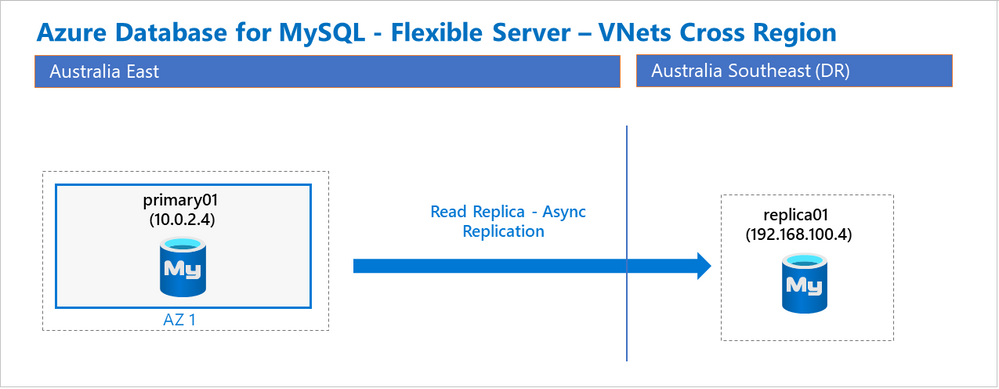
In this illustration, there’s a single Primary server located in Australia East and a Replica is hosted in Australia Southeast. With this setup, it’s important to understand some implementation details, especially around networking and guidance:
- Each server is deployed using the Private Access option.
- Each server is registered to the same Azure Private DNS Zone, in this case, myflex.private.mysql.database.azure.com.
- Each server is on separate a VNet, and the two VNets are peered with each other.
- Each VNet is linked to the Private DNS zone.
The server name, IP address, server type, and region for the two servers I created are shown in the following table:
Server / Service name
|
IP address
|
Role
|
Region
|
primary01.mysql.database.azure.com
|
10.0.2.4
|
Primary
|
Australia East
|
replica01.mysql.database.azure.com
|
192.168.100.4
|
Replica
|
Australia Southeast
|
Note: For more information about Azure Database for MySQL connectivity and networking, see the article Connectivity and networking concepts for Azure Database for MySQL – Flexible Server.
When configured properly, the Private DNS Zone (should appear as shown in the following image:
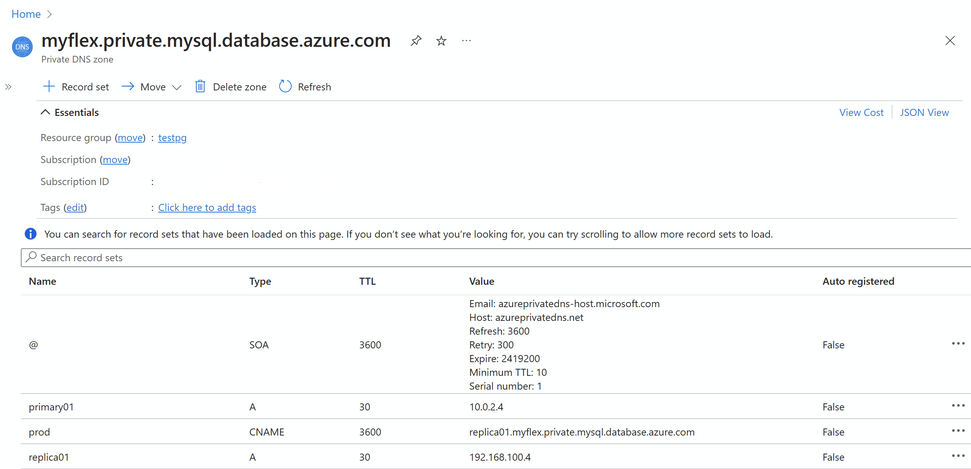
It’s possible to resolve these DNS names from within either VNet. For example, the Linux shell shows the following detail for a Linux VM, which happens to be on the Australia East VNet, and it can resolve the both the service name and the private DNS zone name of each of the servers.
Note: This Linux VM is being used simply to host the ‘nslookup’ and ‘mysql’ binaries that we are using in this article:
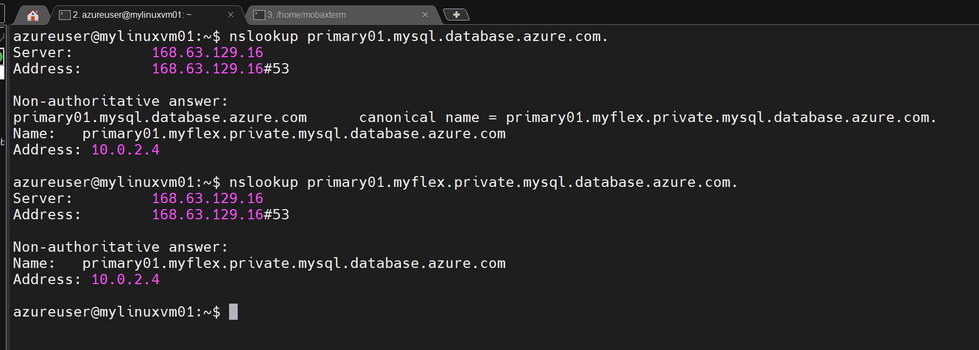
In addition to name resolution and courtesy of our VNet peering, I can also connect to both databases using either the service name or the private DNS name. Running the command-line application ‘mysql’, I’ll connect to the primary server using both DNS names as shown in the following image:
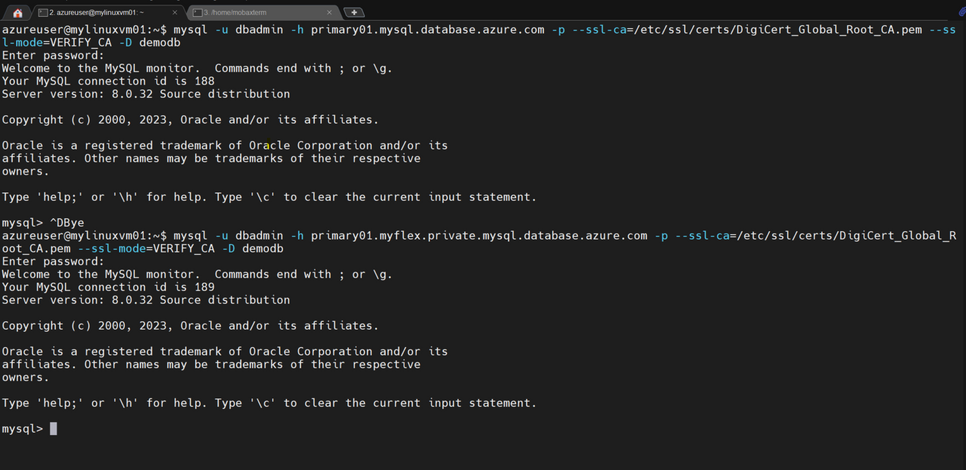
And next, I’ll use ‘mysql’ again to connect to both DNS names for the replica server:
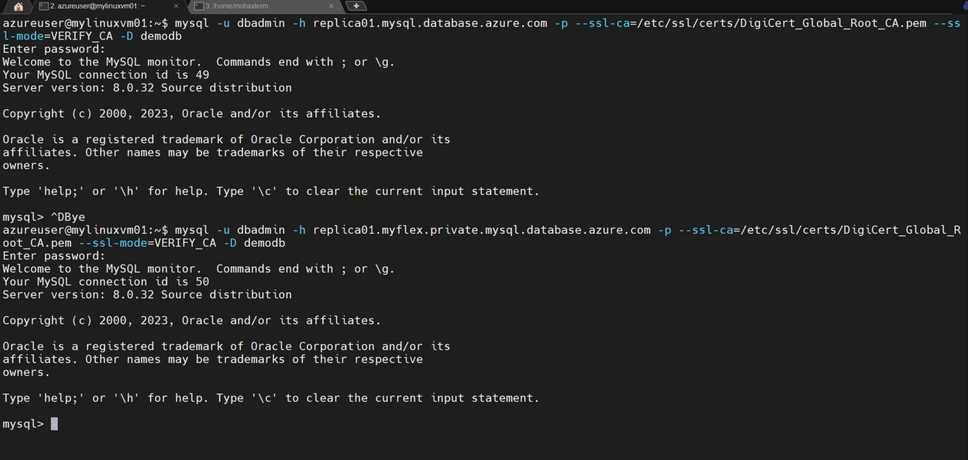
To recap, we have set up a primary server in one region and replica service in another region using the Private Access networking, standard VNET peering, and Private DNS Zone features. I then verified that I could connect to both databases using the service name, or the name allocated by the Private DNS zone. The remaining question, however, is how to failover to the replica database, for example in a DR drill, and allow my application to connect to the promoted replica without making any changes to the application configuration? The answer, it turns out, is pretty simple…
In addition to typical DNS record types of ‘A’ Address and ‘PTR’ Pointer, ‘CNAME’ is another useful record type that I can use as an “alias” to effectively point to another DNS entry. Next, I’ll demonstrate how to configure a ‘CNAME’ record to point to either of the databases in our set up.
For this example, I’ll create a CNAME record with value ‘prod’ that points at the ‘A’ record for the Primary server. Inside the Private DNS Zone you can add a new record by choosing ‘+ Record Set’. Then you can add a CNAME record like so:
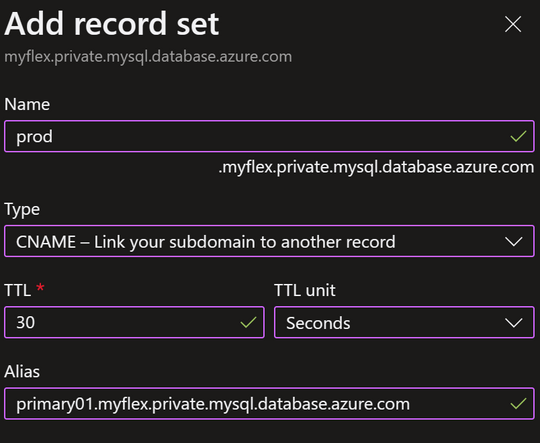
While the default TTL is 1 hour, I’ve reduced this to 30 seconds to limit DNS clients and applications from caching an answer for too long, which can have a significant impart during or after a failover. After I’ve added the CNAME record, the DNS zone looks like this:
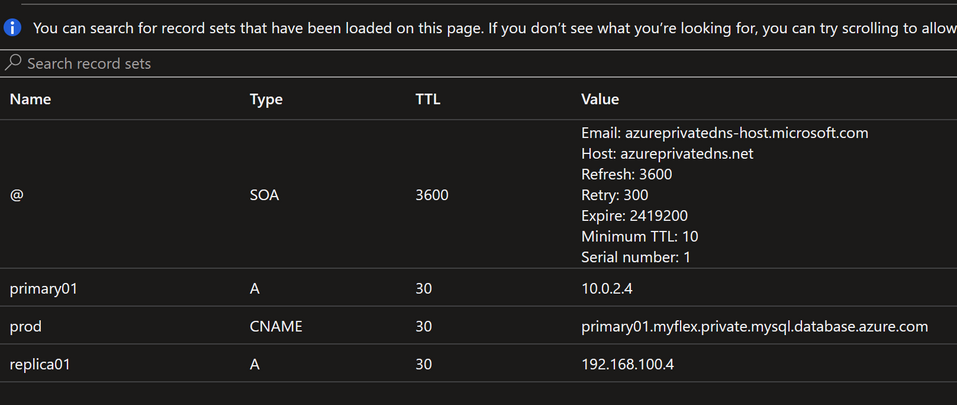
Notice that the new ‘prod’ name points to the ‘A’ record for the primary server.
Now, I’ll verify that I can use the CNAME record to connect to the primary database:
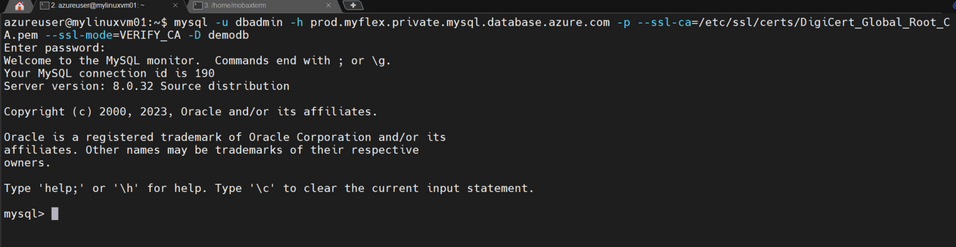
Cool! That’s just DNS doing its thing with the CNAME record type.
It is also possible to edit the CNAME DNS record to point it to the replica:
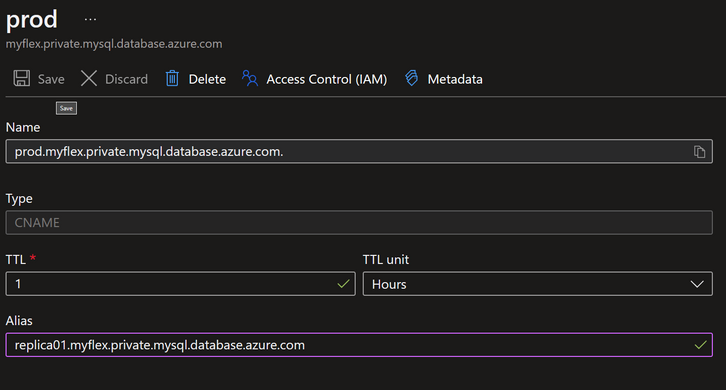
After saving the updated CNAME, when I connect to ‘prod’, it is now connecting to the replica, which is in READ-ONLY mode. I can verify this by trying a write operation, such as creating a table:
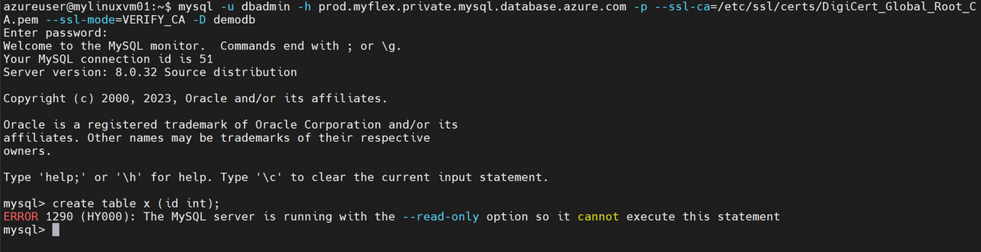
Sure enough, the CNAME ‘prod’ now points to the replica, as expected.
Given what I’ve shown so far, it’s clear the using the flexibility of Azure Private DNS and CNAME records is ideal for this use case.
The last step in this process is to perform the failover and complete the testing.
In the Azure portal, navigate to the Replication blade of either the Replica server or the Standby server, and then ‘Promote’ the Replica:
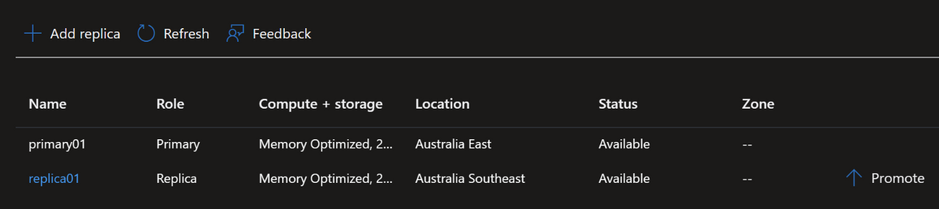
After selecting Promote, the following window appears:
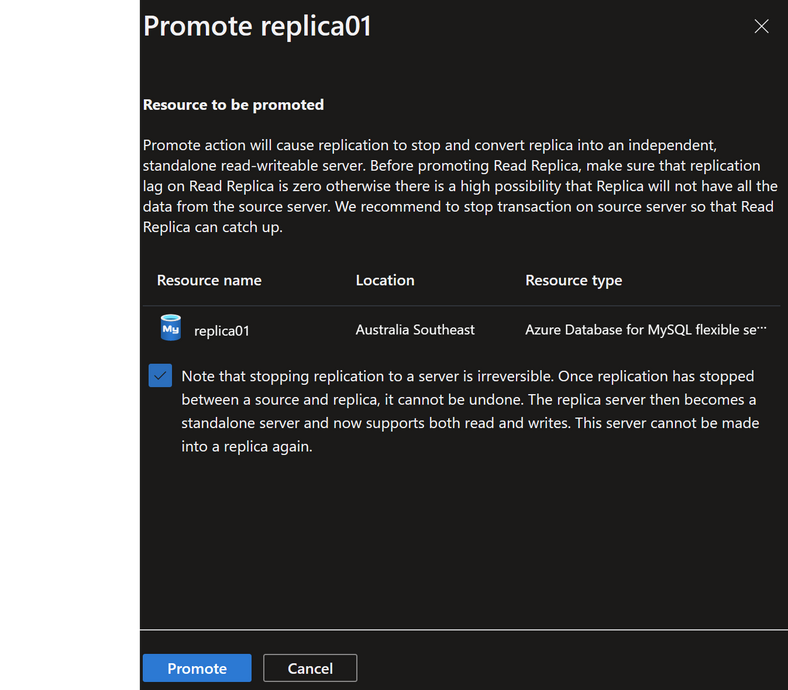
When the newly promoted Replica server is available, I want to verify two things, that the:
- CNAME record points to the Replica (now Primary)
- Database is writeable
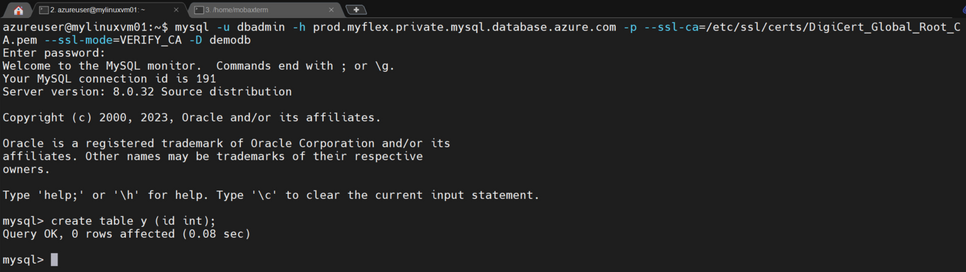
From an application perspective (the application is the mysql client in this article), we haven’t had to make any changes to connect to our database regardless of which region is hosting the workload. This method can be easily integrated within DR procedures or failover testing. Making use of the Azure CLI to semi-automate these changes is also possible and could possibly reduce the likelihood of human errors associated with changing DNS records. However, DNS changes are, in general, less risky than making application configuration changes.
If you have any feedback or questions about the information provided above, please leave a comment below or email us at AskAzureDBforMySQL@service.microsoft.com. Thank you!
by Contributed | Apr 25, 2023 | Technology
This article is contributed. See the original author and article here.
Announcing SynapseML v0.11. The new version contains many new features to help you build scalable machine learning pipelines.
We are pleased to announce SynapseML v0.11, a new version of our open-source distributed machine learning library that simplifies and accelerates the development of scalable AI. In this release, we are excited to introduce many new features from the past year of developments well as many bug fixes and improvements. Though this post will give a high-level overview of the most salient new additions, curious readers can check out the full release notes for all of the new additions.
OpenAI Language Models and Embeddings
A new release wouldn’t be complete without joining the large language model (LLM) hype train and SynapseML v0.11 features a variety of new features that make large-scale LLM usage simple and easy. In particular, SynapseML v0.11 introduces three new APIs for working with foundation models: `OpenAIPrompt`, ` OpenAIEmbedding`, and `OpenAIChatCompletion`. The `OpenAIPrompt` API makes it easy to construct complex LLM prompts from columns of your dataframe. Here’s a quick example of translating a dataframe column called “Description” into emojis.
from synapse.ml.cognitive.openai import OpenAIPrompt
emoji_template = """
Translate the following into emojis
Word: {Description}
Emoji: """
results = (OpenAIPrompt()
.setPromptTemplate(emoji_template)
.setErrorCol("error")
.setOutputCol("Emoji")
.transform(inputs))
This code will automatically look for a database column called “Description” and prompt your LLM (ChatGPT, GPT-3, GPT-4) with the created prompts. Our new OpenAI embedding classes make it easy to embed large tables of sentences quickly and easily from your Apache Spark clusters. To learn more, see our docs on using OpenAI embeddings API and the SynapseML KNN model to create an LLM-based vector search engine directly on your spark cluster. Finally, the new OpenAIChatCompletion transformer allows users to submit large quantities of chat-based prompts to ChatGPT, enabling parallel inference of thousands of conversations at a time. We hope you find the new OpenAI integrations useful for building your next intelligent application.
Simple Deep Learning
SynapseML v0.11 introduces a new Simple deep learning package that allows for the training of custom text and deep vision classifiers with only a few lines of code. This package integrates the power of distributed deep network training with PytorchLightning with the simple and easy APIs of SynapseML. The new API allows users to fine-tune visual foundation models from torchvision as well as a variety of state-of-the-art text backbones from HuggingFace.
Here’s a quick example showing how to fine-tune custom vision networks:
from synapse.ml.dl import DeepVisionClassifier
train_df = spark.createDataframe([
("PATH_TO_IMAGE_1.jpg", 1),
("PATH_TO_IMAGE_2.jpg", 2)
], ["image", "label"])
deep_vision_classifier = DeepVisionClassifier(
backbone="resnet50",
num_classes=2,
batch_size=16,
epochs=2,
)
deep_vision_model = deep_vision_classifier.fit(train_df)
Keep an eye out with upcoming new releases of SynapseML featuring additional simple deep-learning algorithms that will make it easier than ever to train and deploy models at scale.
LightGBM v2
LightGBM is one of the most used features of SynapseML and we heard your feedback on better performance! SynapseML v0.11 introduces a completely refactored integration between LightGBM and Spark, called LightGBM v2. This integration aims for high performance by introducing a variety of new streaming APIs in the core LightGBM library to enable fast and memory-efficient data sharing between spark and LightGBM. In particular, the new “Streaming execution mode” has a >10x lower memory footprint than earlier versions of SynapseML yielding fewer memory issues and faster training. Best of all, you can use the new mode by just passing a single extra flag to your existing LightGBM models in SynapseML.
ONNX Model Hub
SynapseML supports a variety of new deep learning integrations with the ONNX runtime for fast, hardware-accelerated inference in all of the SynapseML languages (Scala, Java, Python, R, and .NET). In version 0.11 we add support for the new ONNX model hub, which is an open collection of state-of-the-art pre-trained ONNX models that can be quickly downloaded and embedded into spark pipelines. This allowed us to completely deprecate and remove our old dependence on the CNTK deep learning library.
To learn more about how you can embed deep networks into Spark pipelines, check out our ONNX episode in the new SynapseML video series:
Causal Learning
SynapseML v0.11 introduces a new package for causal learning that can help businesses and policymakers make more informed decisions. When trying to understand the impact of a “treatment” or intervention on an outcome, traditional approaches like correlation analysis or prediction models fall short as they do not necessarily establish causation. Causal inference aims to overcome these shortcomings by bridging the gap between prediction and decision-making. SynapseML’s causal learning package implements a technique called “Double machine learning”, which allows us to estimate treatment effects without data from controlled experiments. Unlike regression-based approaches, this approach can model non-linear relationships between confounders, treatment, and outcome. Users can run the DoubleMLEstimator using a simple code snippet like the one below:
from pyspark.ml.classification import LogisticRegression
from synapse.ml.causal import DoubleMLEstimator
dml = (DoubleMLEstimator()
.setTreatmentCol("Treatment")
.setTreatmentModel(LogisticRegression())
.setOutcomeCol("Outcome")
.setOutcomeModel(LogisticRegression())
.setMaxIter(20))
dmlModel = dml.fit(dataset)
For more information, be sure to check out Dylan Wang’s guided tour of the DoubleMLEstimator on the SynapseML video series:
Vowpal Wabbit v2
Finally, SynapseML v0.11 introduces Vowpal Wabbit v2, the second-generation integration between the Vowpal Wabbit (VW) online optimization library and Apache Spark. With this update, users can work with Vowpal wabbit data directly using the new “VowpalWabbitGeneric” model. This makes working with Spark easier for existing VW users. This more direct integration also adds support for new cost functions and use cases including “multi-class” and “cost-sensitive one against all” problems. The update also introduces a new progressive validation strategy and a new Contextual Bandit Offline policy evaluation notebook to demonstrate how to evaluate VW models on large datasets.
Conclusion
In conclusion, we are thrilled to share the new SynapseML library with you with you and hope you will find that it simplifies your distributed machine learning pipelines. This blog only covered the highlights, so be sure to check out the full release notes for all the updates and new features. Whether you are working with large language models, training custom classifiers, or performing causal inference, SynapseML makes it easier and faster to develop and deploy machine learning models at scale.
Learn more
Recent Comments