by Scott Muniz | Jul 15, 2020 | Azure, Microsoft, Technology, Uncategorized
This article is contributed. See the original author and article here.
One of Azure’s largest online conferences returned even bigger this year as Brazil’s technical community rallied to “uncomplicate” all things cloud.
The fifth edition of Uncomplicating Azure – or Descomplicando o Azure – expanded from one to two weeks thanks to the support of more than 40 expert presentations on Cloud, Dev, Data, AI, IoT, Productivity and more.
The online conference from June 15-26 expanded farther than South America’s largest country to include speakers from 11 countries. So far, the event’s 35 hours of content has received 28,000 views and counting.
MVP and event organizer Rubens Guimarães said the conference sought to connect communities, people, cultures, and knowledge through digital channels in real-time.
“Participating in communities is something that requires effort, constant learning, time, and dedication, but it is very rewarding when colleagues come to you to talk about having earned a new certification through a lecture tip or getting a new job or even solving a problem of project,” Rubens said. “Being part of communities is something that completes my daily life, strengthening me as an individual in society.”
The ability to connect different people of different backgrounds was a particular highlight, Rubens said, with speakers hailing from Russia, India, USA, Argentina, Chile, and other countries. The event’s multi-platform nature enabled for the addition of subtitles in several languages.
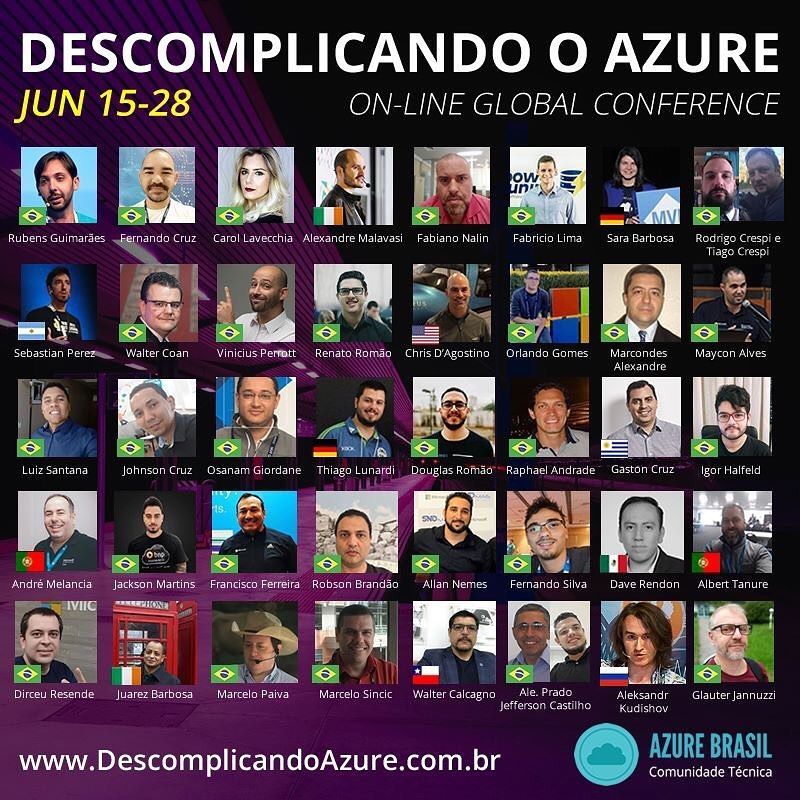
Especially in the context of Brazil’s ongoing battle against the pandemic, the conference served as a meeting ground for members of the technical community to reconnect with each other and the wider world.
“As usual, being part of the Microsoft Community is incredible – and even now with this complicated situation, Brazilians can keep warm and alive through webinars,” said Brazilian Sara Barbosa, who previously served as an MVP for Office Apps & Services before recently joining Microsoft as an FTE. “The energy and content each speaker shared were great. Even though Descomplicando o Azure has a focus on Azure, the diversity of presentations allowed customers, enthusiasts, and community folks to learn about a variety of Microsoft technologies such as SQL, Power Platform, and others.”
Similarly, Brazilian MVP for Data Platform Rodrigo Crespi reinforced the benefit of consistently learning from other like-minds at home and abroad. “Being part of a technical community allows me to meet and interact with the brightest minds in IT,” he said. “There were several important moments, but I highlight my great satisfaction in sharing the little of my knowledge with various people around the world.” Brazilian MVP for Data Platform Fabricio Lima agreed that the event further developed his professional skillset.
Irish-based MVP speaker Alexandre Malavasi praised the sheer breadth and depth of the conference speakers, noting that the diversity of themes meant any audience from students to company managers would find something of use.
“It is extremely rare to see an event that involves so many speakers, including those with international participation, being completely free to the community,” Alexandre said. “I am sure that the event contributed for IT professionals, in general, to understand more accurately the potential, extent, and possibilities that Azure can provide for the development of robust, resilient, high-performance, and cost-effective applications.”
US-based MVP Gaston Cruz said the conference’s international perspective would be useful to many going forward. “This event not only allowed people to learn but also to get a lot of new ideas of how to implement cutting edge technologies and leverage them to support business needs and transform them in the worldwide context that we are in now.”
Argentine-based MVP Sebastián Pérez said he enjoyed the engagement of attendees, with some even following up after his presentation for more information. Meanwhile, German-based MVP Reconnect member Thiago Lunardi said he simply enjoyed being back in the Microsoft community with other thought leaders. “For me, [the highlight] was the minutes before going live. I was out of the spotlight for over 2 years, and the feeling to be back contributing was like I had never left it behind. It’s great to be part of Microsoft community, just great.”
While Uncomplicating Azure is an annual event, Rubens said the organizing team was already looking for an adaptation to be held every six months. Check out the YouTube channel to watch presentations from the conference.
by Scott Muniz | Jul 15, 2020 | Azure, Microsoft, Technology, Uncategorized
This article is contributed. See the original author and article here.
AI and Machine Learning techniques are changing the ways industries process data. And one of the most exciting developments is the ability to process at the edge, next to cameras, sensors, or other systems generating that data. This allows you to get insights right away, without needing to send everything to the cloud first – saving you bandwidth and getting results faster.
We designed Azure Stack Edge for exactly these situations. It’s an extension of the Azure cloud that lets you run analysis locally, but still controlled and managed from the cloud. So, you can deploy and monitor from the cloud, but have everything running at your site, right where your data is generated. Whether that’s a grocery store improving operations, a hospital improving efficiency in the Operating Room, or cities looking to improve traffic safety and efficiency.
One of the cool things about Azure Stack Edge is you can install it, and then have it analyze data from your existing systems. This opens up all sorts of new opportunities you might not expect. For example, airports have big existing scanners checking luggage for dangerous items. But as part of conservation efforts, they also want to check for illegal animal parts. This can reduce poaching and improve environment sustainability. With Azure Stack Edge you have those scanners do double duty. They can continue to do their normal work looking for dangerous items, and also send the scans to Azure Stack Edge to run AI models designed to detect other items. There’s a new Microsoft Mechanics video that highlights this use case and how it uses Azure Stack Edge as a platform to run locally. Definitely check out the video to get the full story.
If your business is looking to do AI analysis at the edge, Azure Stack Edge is a great solution. It’s part of Azure, so there’s nothing to buy. You sign up like any other Azure service and we send you a server and bill you monthly on your normal Azure bill. It has built in ML acceleration hardware, and works with Microsoft’s container deployment systems. Read more about Azure Stack Edge here, or order one from the Azure Portal.
by Scott Muniz | Jul 15, 2020 | Azure, Microsoft, Technology, Uncategorized
This article is contributed. See the original author and article here.
High-performance computing (HPC) can be expensive and complex to set up and use efficiently. Traditionally, HPC has been restricted to academia, research institutes, and enterprises with the capacity to operate large datacenters. Furthermore, it has always been a challenge to deliver optimized HPC systems with complex workflows involving different applications in a user-friendly and accessible environment. Dev Subramanian, CTO at Drizti Inc., elaborates on the challenges and describes how the Toronto startup aims to democratize HPC with Microsoft Azure:
Even enterprises that can afford to build massive datacenters can deliver HPC from only a few locations, limiting the availability of HPC for a globally distributed workforce. Additionally, applications requiring HPC generate a lot of data, and a centrally located datacenter increases the strain on networks.
These inherent complexities have limited the use of HPC across industries, slowing down cutting-edge innovation and leading organizations to investigate ways to make HPC more accessible and easier to use.
Drizti offers a cloud-based HPC platform for “personal supercomputing.” The HPCBOX platform on Microsoft Azure Marketplace delivers turnkey vertical solutions with optimized HPC for accelerating innovation in advanced manufacturing, machine learning, artificial intelligence, and finance. Drizti’s mission is to make supercomputing technology accessible on the PC of every scientist, developer, and engineer, wherever they are and whenever they need it.
With 58 global regions and availability in 140 countries, Azure is one of the few public cloud infrastructure providers focused on implementing HPC capability in the cloud.
Various Azure instance sizes are built for handling HPC workloads and are offered alongside high-speed RDMA InfiniBand networking and high-performance file systems. Furthermore, the rich set of APIs that Azure exposes enables HPC to be combined with other services, and this is what Drizti has done with its HPCBOX platform.
HPCBOX user interface
HPCBOX delivers a fully interactive, workflow-enabled HPC platform for automating complex application pipelines. It offers a PC-like user experience and delivers various turnkey vertical solutions optimized for applications such as ANSYS, Simcenter STAR-CCM+, Siemens Nastran, Abaqus, Horovod, SU2, OpenFOAM, and many others that can benefit from big compute on Azure. The HPCBOX platform uses big compute capabilities Azure offers, including state-of-the-art HB2, HB, HC, H, N, and NC-Series instance sizes.
Through the reach of the Azure Marketplace, HPCBOX delivers turnkey HPC solutions directly within an end user’s subscription, whether it is an EA, web-direct, or CSP subscription. This approach lets users access HPC for their applications within minutes of deploying an HPCBOX cluster while remaining compliant with all the corporate policies set by their organization. Furthermore, by delivering the HPCBOX solution directly within a user’s subscription, Drizti can make sure all the data resides within the user’s subscription and in their region of choice.
Finally, being a Microsoft co-sell certified solution makes HPCBOX fully compatible with Azure services and infrastructure, giving users confidence they can utilize HPCBOX on Azure to dramatically cut costs, get significantly faster results, and spend more time on innovation rather than system setup and configuration.
To learn more about HPCBOX on Azure, watch this video and sign up for a personal supercomputing promo.
by Scott Muniz | Jul 14, 2020 | Azure, Microsoft, Technology, Uncategorized
This article is contributed. See the original author and article here.
In the past few months, the CLI team has been working diligently to improve the in-tool user experience (UX) for you. We listened to your feedback and designed the features so that they could be useful and applicable the moment you start interacting with the tool.
In the following article, we will share with you our latest updates on performance improvement on command execution and configurable output with the “only-show-errors” tag.
Significant performance improvements on client-side command execution
One top frustration we’ve been hearing from both our new and experienced CLI users is that the speed of command execution could use some improvement. Our team recognized this challenge and immediately followed up with optimizations. And the result was phenomenal! Every client-side command is now 74% faster.
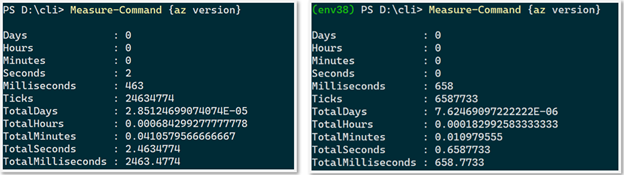
Figure 1: Comparison of the performance of client side command execution, before vs. after
Interruption free automation with “–only-show-errors” flag:
For those of you who’d been frustrated with Azure CLI’s warning messages endlessly interrupting your ongoing scripts, we’re delighted to share with you a simple resolution! Our team recently released the “–only-show-errors” flag which enables you to disable all non-error output (i.e warnings, info, debug messages) in the stderr stream. This has been highly requested by our DevOps and Architect fans and we hope you can take advantage of this as well. We support the feature on both the per command basis and also via direct updates in the global configuration file. This way you could easily leverage the feature either interactively, or for scripting/automation purposes
To enable it, simply append —only-show-errors to the end of any az command. For instance:
az timeseriesinsights environment list —only-show-errors
Below is an example of before vs. after of enabling the flag. We can see that the experimental message has been suppressed:

Fun fact: our team has actually considered the verbiage around “—no-warnings” and “—quiet” but eventually settled on “—only-show-errors” given its syntactical intuitiveness.
Call to action:
Similar to last time, some of these improvements are early in the preview or experimental stage but we certainly do look forward to improving them to serve you better. If you’re interested, here is where you can learn more.
We’d love for you to try out these new experiences and share us your feedback on their usability and applicability for your day-to-day use cases. Also please don’t hesitate to share us any ideas and feature requests on how to improve your overall in tool experience!
Stay tuned for our upcoming blogs and subscribe to it ! See you soon
by Scott Muniz | Jul 14, 2020 | Azure, Microsoft, Technology, Uncategorized
This article is contributed. See the original author and article here.
Data science is about extracting knowledge from data. Data science is an important area of study because it is a tool that data scientists leverage to gain insights from data and prepare it for the machine learning modeling phase. By “doing data science”, data scientists actually apply techniques, such as data pre-processing and cleaning, feature engineering and descriptive statistics, to their data in order to understand it and start building AI solutions.
In this sense, data science has become an area of study that universities and companies should look at as a first step to start their machine learning journey:

To learn more about Machine Learning versus AI and Deep Learning, please visit: https://www.aka.ms/DLvsML
Sarah Guthals, PhD (@sarahguthals) and Francesca Lazzeri, PhD (@frlazzeri) have released “A Developer’s Introduction to Data Science”, a 28-part video series that focuses on how to use data science to build machine learning solutions: this series is now live on both Channel 9 and YouTube.
The content of this series is structured as follow:
Video Title
|
Video Description
|
Introduction to the Developer’s Intro to Data Science Video Series
|
In this 28-video series, you will learn important concepts and technologies to build your end-to-end machine learning applications on Azure. To learn more, check out: http://www.aka.ms/DevIntroDS_GitHub and http://www.aka.ms/DevIntroDS_Learn
|
What is the Data Science Lifecycle?
|
In this video you will learn what the Data Science Lifecycle is and how you can use it to design your data science solutions.
|
How do you define your business goal and scope your data science solution?
|
In this video, Sarah describes the problem that she is facing where she thinks data science methods might be able to help her improve her business goals.
|
What is Machine Learning?
|
What is Machine Learning? In this video you will learn what machine learning, supervised learning and unsupervised learning are and how you can use the model development cycle to build, train, test and deploy your machine learning models.
|
Which Machine Learning Algorithm Should You Use?
|
Which machine learning algorithm should I use? In this video you will learn how to select the right machine learning algorithm for your data science scenario and how to answer different questions with different machine learning approaches.
|
What is AutoML?
|
In this video, you will learn how you can use Automated machine learning (Automated ML) to accelerate the data science life cycle.
|
How do you create a machine learning resource in Azure?
|
In this video, you will learn how to create a Machine Learning resource inside of Azure. By using Azure for your machine learning toolset, you’re able to create the storage account, application insights, key vault and container registry (all resources that will support your machine learning work) in just a matter of minutes.
|
How do you setup your local environment for data exploration?
|
In this video, you will learn how to setup your local environment for data exploration. Specifically, you will setup Visual Studio Code to be able to run Python Jupyter Notebooks and connect to your Azure Machine Learning resource.
|
How do Jupyter notebooks work in Visual Studio Code?
|
In this video, you will get an introduction to how Jupyter Notebooks work inside of Visual Studio Code and install the Python packages useful for this data science project, and make sure you have access to the AzureML SDK.
|
How do you connect your Azure Machine Learning resources to your local Visual Studio Code environment?
|
In this video, you will learn how to connect the Machine Learning resource that you created in Azure to your local Visual Studio Code environment. This allows you to run your machine learning experiments on the cloud instead of locally.
|
How do you prepare your data for a time series forecast?
|
In this video you will learn how to prepare your data to be effectively run through machine learning algorithms. Then, you will learn how to upload your data from your local computer into your Azure Machine Learning resource (specifically the datastore resource) and how to
|
Why do you split data into testing and training data in data science?
|
In this video, you will learn why you split your data into training and testing data. Then you will learn how to actually split your data using a date into two different Pandas DataFrames using Python in Visual Studio Code.
|
What is an AutoML Config file?
|
In this video you will learn how to run a machine learning experiment with Automate ML and how to create your AutoMLConfig file to submit an automated ML experiment in Azure Machine Learning.
|
What should your parameters be when creating an AutoML Config file?
|
See how Sarah and Francesca configure and run an AutoMLConfig file to submit an automated ML experiment in Azure Machine Learning.
|
How do you create an AutoML Config file and run your data science experiments on the cloud?
|
In this video, you will actually put your data through AutoML in Azure to train and test with a number of machine learning algorithms that Azure supports.
|
What is Azure Machine Learning?
|
Azure Machine Learning is a cloud-based environment that you can use to train, deploy, automate, manage, and track your machine learning models.
|
How can you collaborate on Jupyter Notebooks using Azure Machine Learning studio?
|
In this video, you will see the Azure Machine Learning Studio and learn how create a Jupyter Notebook in the cloud. By doing this, you ensure you have access to your code anywhere.
|
How do you choose the best model and perform feature engineering?
|
In this video you will learn how to use Automated ML to select your best model and perform features engineering: Automated ML is the process of automating the time consuming, iterative tasks of machine learning model development and can help you optimize when developing end to end applications on Azure.
|
How do you use Azure ML for best model selection and featurization?
|
During training, the Azure Machine Learning service creates a number of in parallel pipelines that try different algorithms and parameters. When configuring your experiments, you can enable the advanced setting featurization, that can help you with automatic data cleansing, preparing, and transformation to generate synthetic features.
|
How do you evaluate and retrieve a time series forecast from Azure Machine Learning?
|
In this video, you will learn how to use an external python function to run your data through a forecast evaluation. Using Python files uploaded to the cloud environment within the Azure Machine Learning Studio, you can call functions within those files from the Jupyter Notebooks within the same cloud environment.
|
How do you score your machine learning model on accuracy?
|
In this video, you will use the root mean squared error, mean absolute error, and mean absolute percentage error to score the accuracy of your model. You will then learn how to visualize the productions of your model within the Jupyter Notebook within the Azure Machine Learning studio cloud environment using scatter plots.
|
How do you deploy a machine learning model as a web service within Azure?
|
In this video, you will gather all of the important pieces of your model to be able to deploy it as a web service on Azure so that your other applications can call it on the fly.
|
What have you learned from deploying a machine learning model as a web service?
|
In this video, Sarah summarizes all of the learnings from measuring the accuracy of the machine learning model used in this series. Sarah also revisits the business goal to determine whether the effort would actually provide valuable information for her business.
|
What is the importance of model deployment in machine learning?
|
In this video, Francesca summarizes the most important steps to deploy your machine learning models with Azure Machine Learning. Model deployment is the method by which you integrate a machine learning model into an existing production environment.
|
How do you select the right machine learning algorithm?
|
A common question in data science is “Which machine learning algorithm should I use?”. In this video you will learn how the algorithm you select depends primarily on two different aspects of your data science scenario: 1) What you want to do with your data? Specifically, what is the business question you want to answer by learning from your past data? 2) What are the requirements of your data science scenario? Specifically, what is the accuracy, training time, linearity, number of parameters, and number of features your solution supports?
|
How does ethics play a role in data science?
|
In this video, Sarah challenges you to think about where ethics plays a role in all data science problems. Regardless of the type of data analysis or machine learning model you are using, your questions, data, and parameters to your algorithms might introduce bias and actually cause harm.
|
What is model interpretability and how can you incorporate it into your data science solutions?
|
Interpretability is critical for data scientists, auditors, and business decision makers alike to ensure compliance with company policies, industry standards, and government regulations. In this video you will learn how to use the Model Interpretability toolkit to explain your models
|
Concluding the Developer’s Intro to Data Science Video Series
|
In this 28-video series, you learnt important concepts and technologies to build your end-to-end machine learning applications on Azure: Sarah and Francesca guided you through the data science process, from understanding your data, to applying machine learning algorithms and deploying your models on Azure.
|
Additional resources:
More videos coming:
The month of July 2020 is Data month for the Microsoft Reactors global live streams on Twitch! For the middle two weeks of July, you will get to dive even deeper on these and similar concepts with Sarah, Francesca, and other Cloud Advocates and Microsoft employees!
by Scott Muniz | Jul 14, 2020 | Azure, Microsoft, Technology, Uncategorized
This article is contributed. See the original author and article here.
We are delighted to introduce the Public Preview for the Anomalous RDP Login Detection in Azure Sentinel’s latest machine learning (ML) Behavior Analytics offering. Azure Sentinel can apply machine learning to Windows Security Events data to identify anomalous Remote Desktop Protocol (RDP) login activity. Scenarios include:
- Unusual IP – the IP address has rarely or never been seen in the last 30 days.
- Unusual geolocation – the IP address, city, country, and ASN have rarely or never been seen in the last 30 days.
- New user – a new user logs in from an IP address and geolocation, both or either of which were not expected to be seen based on data from the last 30 days.
Configure anomalous RDP login detection
- You must be collecting RDP login data (Event ID 4624) through the Security events data connector. Make sure that in the connector’s configuration you have selected an event set besides “None” to stream into Azure Sentinel.
- From the Azure Sentinel portal, click Analytics, and then click the Rule templates tab. Choose the (Preview) Anomalous RDP Login Detection rule, and move the Status slider to Enabled.
As the machine learning algorithm requires 30 days’ worth of data to build a baseline profile of user behavior, you must allow 30 days of Security events data to be collected before any incidents can be detected.
Recent Comments