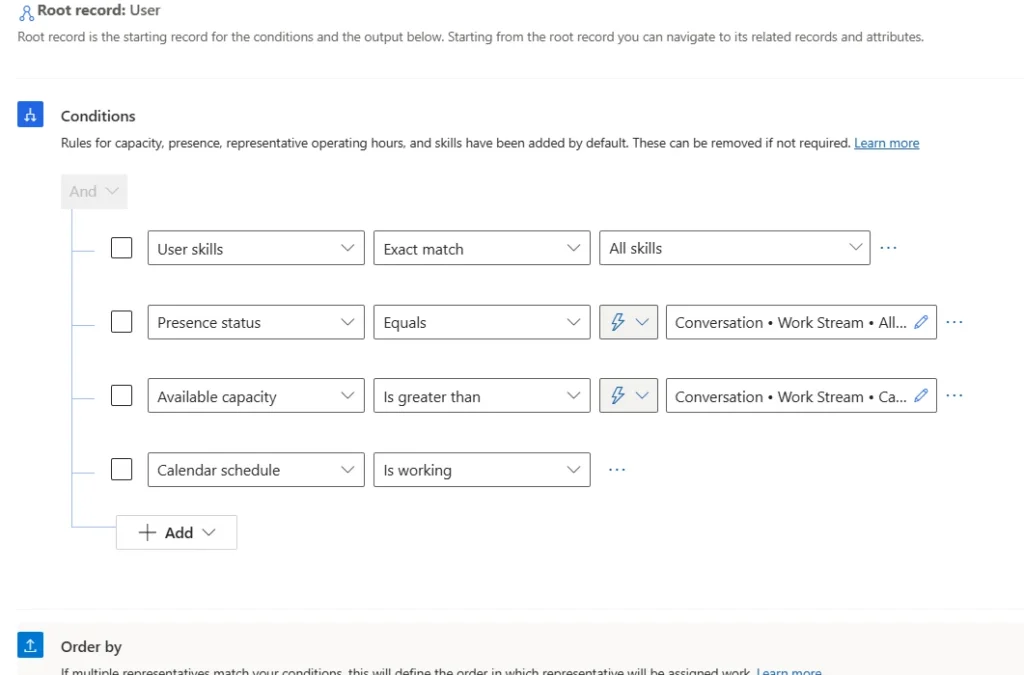
Use least active routing on messaging channels
This article is contributed. See the original author and article here.
In customer service, efficient workload distribution is vital for high customer satisfaction and operational efficiency. Unified routing capabilities in Dynamics 365 Customer Service and Dynamics 365 Contact Center help organizations continuously fine-tune their operations. Least active routing assigns work items based on when service representatives finish their last conversation. Initially used for voice channels, this strategy is now extending to messaging channels, with the additional ability to factor in the load on a service rep, based on the concurrent conversations being handled.
What is least active routing?
Least active routing fairly distributes tasks among service representatives by considering last capacity release time. This ensures adequate rest between tasks, better utilization, improved customer satisfaction, as well as better customer service representative satisfaction.
Enhancements to least active routing
The extension of least active routing to messaging channels brings two significant enhancements. First, it has the ability to factor in the concurrent workload of a service representative. And second, it considers the workload and conversation completion across voice and messaging channels. These enhancements ensure fair and efficient workload distribution in a blended workforce handling multiple channels and individual service reps handling multiple conversations at the same time.
Least active routing in action
Contoso Health is a multinational healthcare and insurance provider. It has a large customer support organization covering more than 20 product lines, handled by more than 5,000 service representatives worldwide. Customers either call the contact center or use the chat option on the website to get their queries resolved.
In the contact center, a service representative can talk to only one customer at a time while engaging with up to three customers over chat in parallel. Eugenia, the director of customer support at Contoso, observes that some of her service representatives are being utilized up to 95% in their schedule. Meanwhile, others are occupied only for only 70-75%, and she wants to solve this problem. While doing that, she also wants to make sure not to impact the key metrics like customer satisfaction and SLAs. She comes across the least active routing assignment method and tries it for voice and messaging queues.

Scenario 1: Different workloads across service reps
Oscar Ward and Victoria Burke are two service representatives with the same skills. Oscar works on the Members Messaging queue, while Victoria works on Members Messaging and Returns Voice queues.
- Number of conversations with Oscar: 1 chat
- Number of conversations with Victoria: 1 call and 1 chat
At 1:00 PM, a new chat conversation arrives.
Because Oscar has fewer concurrent conversations than Victoria, the new chat is assigned to Oscar.
Scenario 2: Equal workloads across service reps
Maya and Hailey are two service representatives with the same skills. Maya works on the messaging queue for Orders, while Hailey works on the messaging queue for Orders and Voice queue for Delivery queries.
Let’s assume that Hailey is working on a call and chat at the same time while Maya is engaged in two chat conversations.
Maya completes one of the chats at 1:55 PM, and Hailey completes the chat at 2:00 PM. A new chat conversation arrives at 2:05 PM.
- Number of conversations with Hailey: 1 call
- Number of conversations with Maya: 1 chat
Because both Maya and Hailey have the same number of active assignments, the least active assignment strategy considers the last capacity release time across both voice and messaging queues.
Maya is determined to be least active compared to Hailey and therefore, the new chat is assigned to Maya.
Note: Least active routing can also be used as an Order by condition in the custom assignment methods.
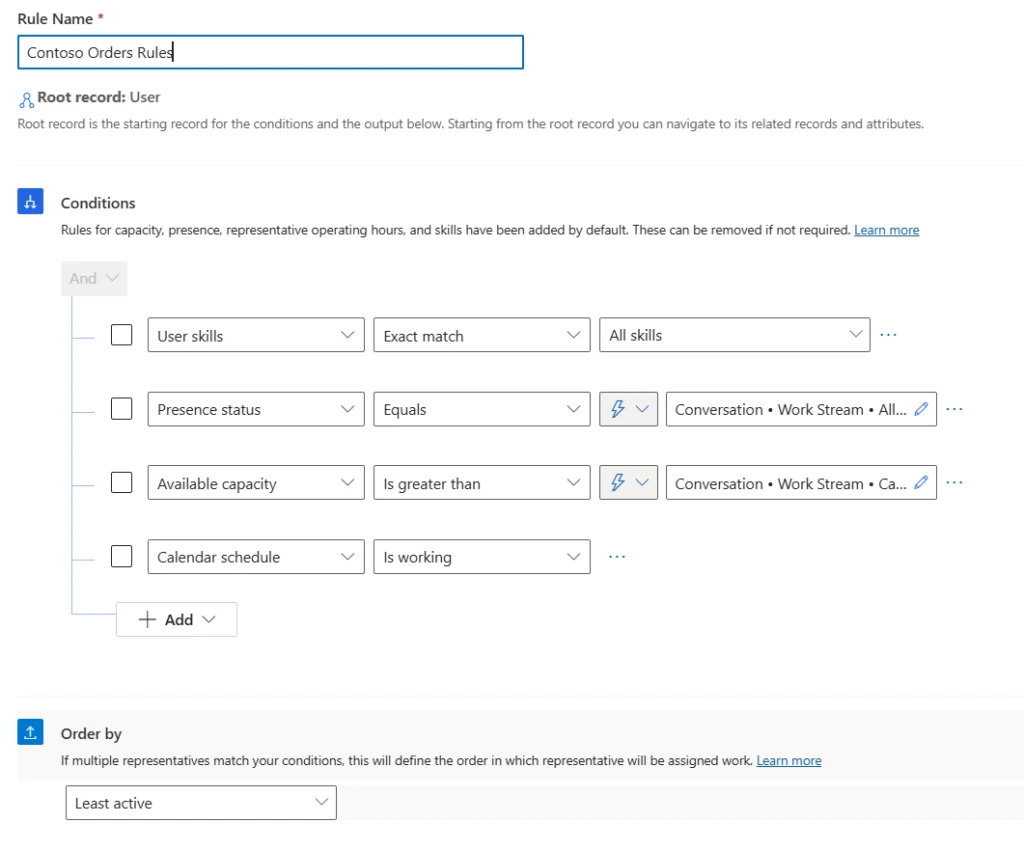
Build custom reports to monitor an agent’s last capacity release time
The least active assignment method works based on when the agent ended his or her last conversation. This data about the agent’s last call end time or last capacity release time, is available in the Dataverse entity ‘msdyn_agentchannelstateentity’. Organizations can use the model customization feature in Dynamics 365 Customer Service to build a custom report that provides a view of this data.
Learn more
To learn more about least active routing, read the documentation: Assignment methods for queues | Microsoft Learn
Related articles:
The post Use least active routing on messaging channels appeared first on Microsoft Dynamics 365 Blog.
Brought to you by Dr. Ware, Microsoft Office 365 Silver Partner, Charleston SC.
Recent Comments